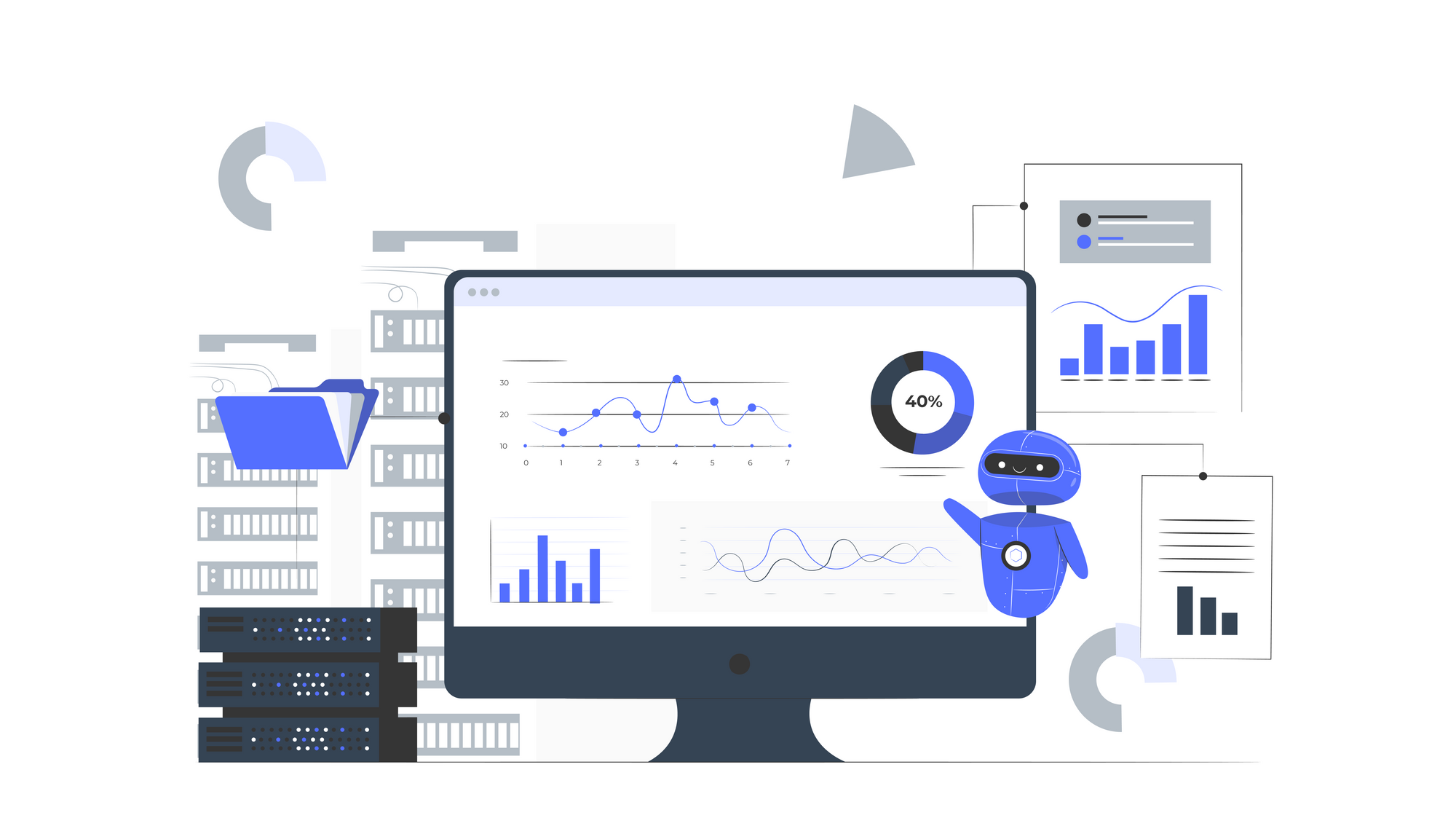
In the modern data-driven landscape, 80-90% of an organization's data is unstructured — a goldmine of potential insights hidden in plain sight within texts, videos, and social media.
Yet, in a staggering disconnect, Deloitte's findings reveal that only 18% of companies have efficiently extracted value from this uncharted digital territory. The untapped potential speaks volumes, but the capacity to extract and process unstructured data into actionable intelligence remains challenging for many.
Let's dive in and explore how to get your business to join that 18% and make the most of unstructured data.
What is unstructured data?
Any information not arranged into any sequence, scheme, or specific structure that makes it easy to read for others is called unstructured data.
Unstructured data is the raw, disorganized information that floods our digital ecosystem daily. From emails and images to social media interactions and beyond, it defies conventional data models, presenting unique business challenges and opportunities.
Processing and leveraging unstructured data requires innovative tools like AI-enhanced OCR (Optical Character Recognition) that convert unorganized information into clarity.
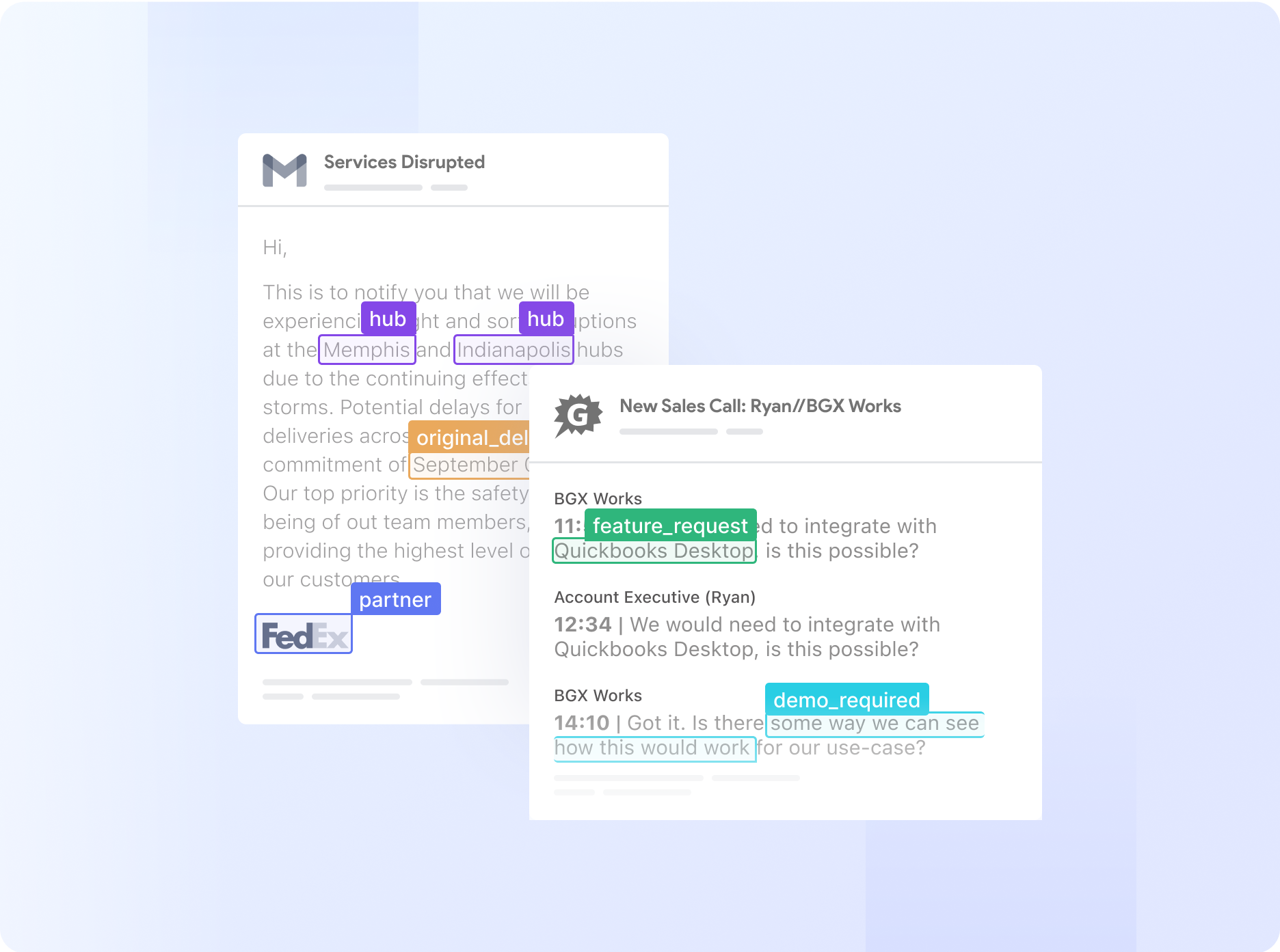
Extracting insights from unstructured data with Nanonets
Nanonets takes the heavy lifting out of processing unstructured data with its AI, ML, and NLP capabilities. This way, you can automate the data extraction process, transforming large volumes of unstructured information into actionable insights.
Here's a straightforward guide to help you get started with unstructured data extraction:
Step 1: Gather your data
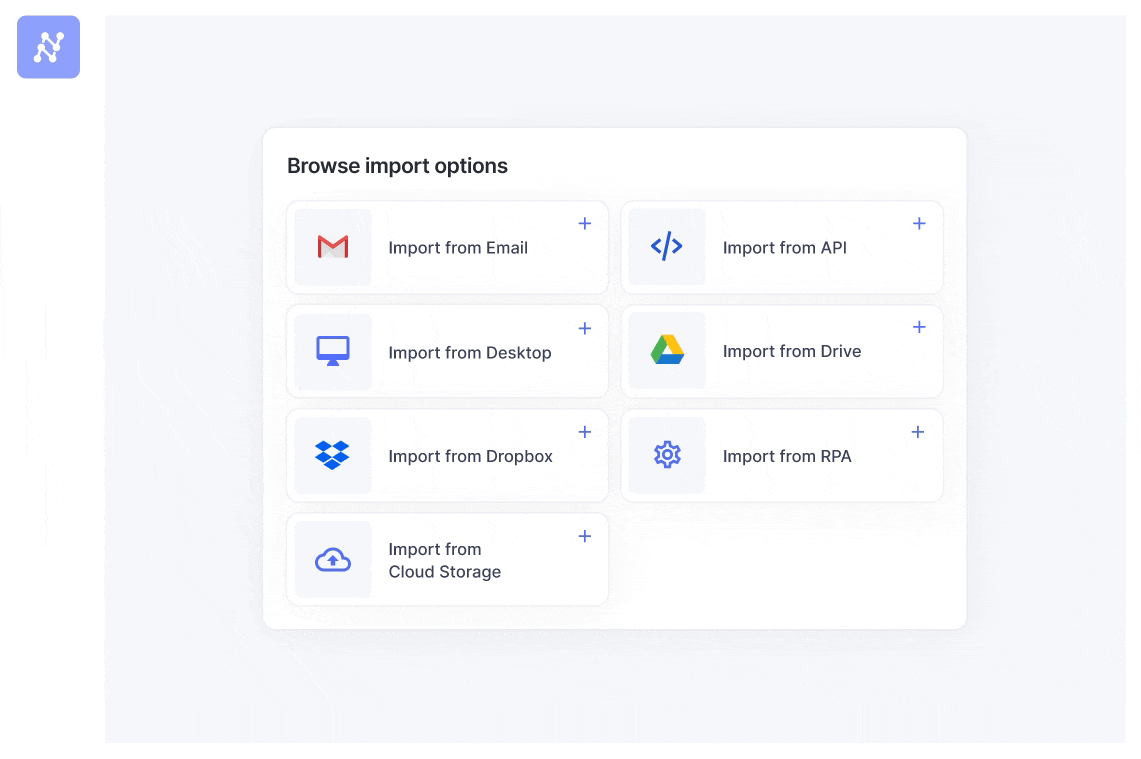
Collect the unstructured data that requires analysis — be it images, text files, PDFs, videos, or audio files—that you want to make sense of.
Step 2: Upload to Nanonets
Head over to the Nanonets website and upload all the collected data. Don’t have an account yet? No problem! Set one up in a snap. You can upload files manually or in bulk from your Google Drive, Dropbox, or SharePoint. You can even use the auto-import options or APIs to import data into the system seamlessly.
Step 3: Select or customize your model
Choose an appropriate OCR model from Nanonets’ collection tailored to different document types. You can train a custom OCR model for unique data sets by uploading a few sample sets and tagging the necessary data points.
The intuitive interface makes the model training process straightforward, even for those new to machine learning. The model learns from these samples and becomes more accurate over time, adapting to the specific nuances of your data.
Step 4: Data processing
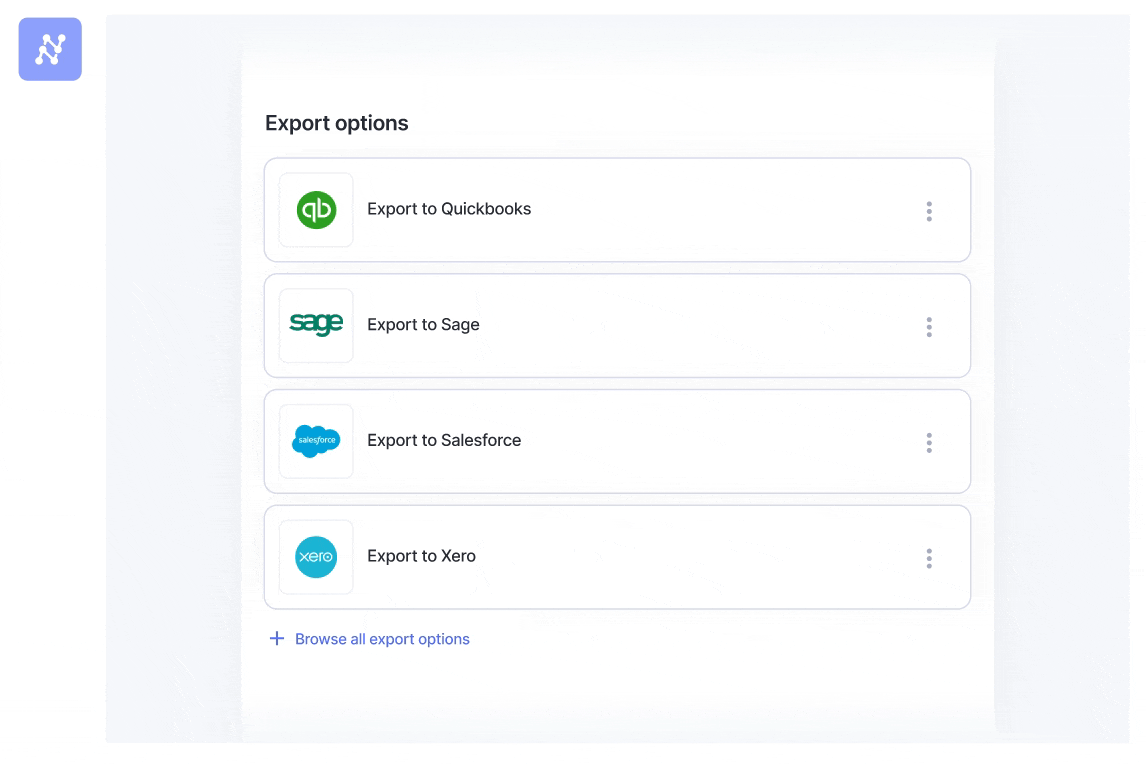
Deploy your selected or trained model on the uploaded data to begin the extraction process. The model will convert unstructured information into a structured format, such as tables, Excel spreadsheets, or CSV files, enhancing readability and analysis potential. You can use the Zapier integration to connect with other tools and automatically send your processed data to the required platforms for further analysis or reporting.
Step 5: Review and refine
.png)
Check the quality of extracted results. You can easily fine-tune the model using Nanonets' drag-and-drop platform until the desired accuracy is achieved. Continuous improvements and feedback loops mean that your model becomes more efficient and more intelligent with each use, reducing the need for manual intervention.
Step 6: Integration and automation
Leverage the Nanonets integrations with your existing systems, automating the entire workflow. Set up triggers for incoming data to be processed automatically without manual upload. This integration creates a seamless flow from data collection to insight generation, enabling real-time decision-making and enhanced business intelligence.
Step 7: Insight extraction
Utilize the now-structured data to derive valuable business insights, enabling more informed decision-making. Export the organized data for comprehensive analysis as required.
Please note that the process may vary slightly depending on the nature of the unstructured data and the desired insights.
Nanonets streamlines the process with advanced automation workflows, sophisticated OCR technology, and a user-friendly interface. And it is a no-code platform — you won't need any programming experience to complete the task.
What are the examples of unstructured data?
Unstructured data exists in diverse formats throughout our digital environment. These formats include textual, non-textual, human-generated, and machine-generated information, each presenting unique challenges for data processing and analysis.
Understanding these different types helps organizations identify what data they possess and how to extract value from it.
Here’s a snapshot:
1. Text data
The term text data refers to the unstructured information found in emails, text messages, written documents, PDFs, text files, Word documents, and other files.
2. Multi-media messages
Multimedia messages, including images (JPEG, PNG, GIF), audio, or video formats, are unstructured data. These messages have complex codes that do not follow a pattern.
All images, videos, or audio files can be encrypted binary codes that lack structure. As a result, they are considered unstructured data.
For instance, what do you see here?
Although it appears as just unstructured data, it is actually an image of a red car. The data of photos, videos, and audio are not decipherable and require observation to understand, which is why they are classified as unstructured data.

3. Website content
All the websites are filled with any information available in the form of long, scattered, and disorganized paragraphs. This is data with valuable information, but it is still not worthy because the proper composition of data is required.
4. Sensor Data - IoT devices
The Internet of Things is a physical device that collects information about its surroundings and sends the data back to the cloud. IoT devices send back sensitive sensor data, which can be unstructured. Examples of IoT devices sending sensor data could be traffic monitoring devices and music devices like Alexa, Google Home, etc.
5. Business documents
Businesses deal with documents of various types, like PDFs, emails, invoices, customer orders, and more. All the documents have different structures. To extract data from PDF, and other paper-based documents, businesses can use intelligent document processing software like Nanonets.
What is the difference between structured and unstructured data?
Data can be classified into three types: structured, semi-structured, and unstructured. Each type of data has its own unique characteristics and potential uses. Let's dive deeper into their differences.
Structured data: This is data that adheres to a specific format or schema. It is organized to make it easily searchable and storable in relational databases. It typically includes data that can be entered, stored, and queried in a fixed format like spreadsheets or SQL databases.
Unstructured data: Your description here is also correct. Unstructured data doesn't follow a specific format or model and is more complex to manage and interpret. It's often text-heavy but can also be in images, videos, and other media. This type of data is commonly found on social media or within multimedia content, and it's not organized in a pre-defined manner.
Semi-structured data: Semi-structured data is indeed a mix of both types. While it has no rigid structure, it often contains tags or other markers to separate semantic elements and enforce hierarchies of records and fields. Examples include JSON and XML files.
Parameter | Structured Data | Unstructured Data | Semi-structured Data |
---|---|---|---|
Data Model | - Follows a rigid schema with rows and columns - Easily stored in relational databases (RDBMS) |
- Lacks predefined format - Appears as emails, images, videos, etc. - Requires dynamic storage |
- Identifiable patterns and markers (e.g., tags in XML/JSON) - Does not fit into a traditional database structure |
Data Analysis | - Simplifies analysis - Allows straightforward data mining and reporting |
- Requires complex techniques like NLP and machine learning - More effort to interpret |
- Easier to analyze than unstructured data - Recognizable tags aid in analysis |
Searchability | - Highly searchable with standard query languages like SQL - Quick and accurate data retrieval |
- Difficult to search - Needs specialized tools and advanced algorithms |
- Partial organization aids in searchability - Metadata and tags can help |
Visionary Analysis | - Predictive analytics and trend analysis are straightforward due to quantifiable nature | - Rich in qualitative insights for visionary analysis - Requires significant effort to mine |
- Partial organization allows some direct visionary analysis - May need processing for deeper insights |
What are the challenges of working with unstructured data?
While 64% of organizations utilize structured data, a mere 18% are tapping into unstructured data. The significant potential remains largely untapped due to the challenges of processing unstructured data.
The challenges include:
- Bulk and form: Unstructured data is created in high volume and varied forms, creating a complex landscape for staff to manage. This diversity ranges from text documents to multimedia files, requiring considerable time for proper handling.
- Storage demands: As businesses increasingly prioritize digital efficiency, unstructured data takes up more space and is more complicated to manage than its structured counterparts. This can create storage issues and complicate data management.
- Compromised data quality: Sifting through unstructured data to find relevant information is challenging. It often includes nonessential details that obscure valuable insights, necessitating robust filtering to ensure data accuracy and usefulness.
- Extraction time: In a world where speed often equates to competitive advantage, the time-consuming process of extracting usable information from unstructured data may be seen as a hindrance to timely decision-making and operational agility.
Fortunately, AI-powered data extraction tools have made the processing and extraction of unstructured data much more efficient, saving time and effort.0 Nanonets stands out among these with its best-in-class OCR capabilities, intelligent AI-based data extraction models, and automated workflows that enhance productivity while ensuring accuracy.
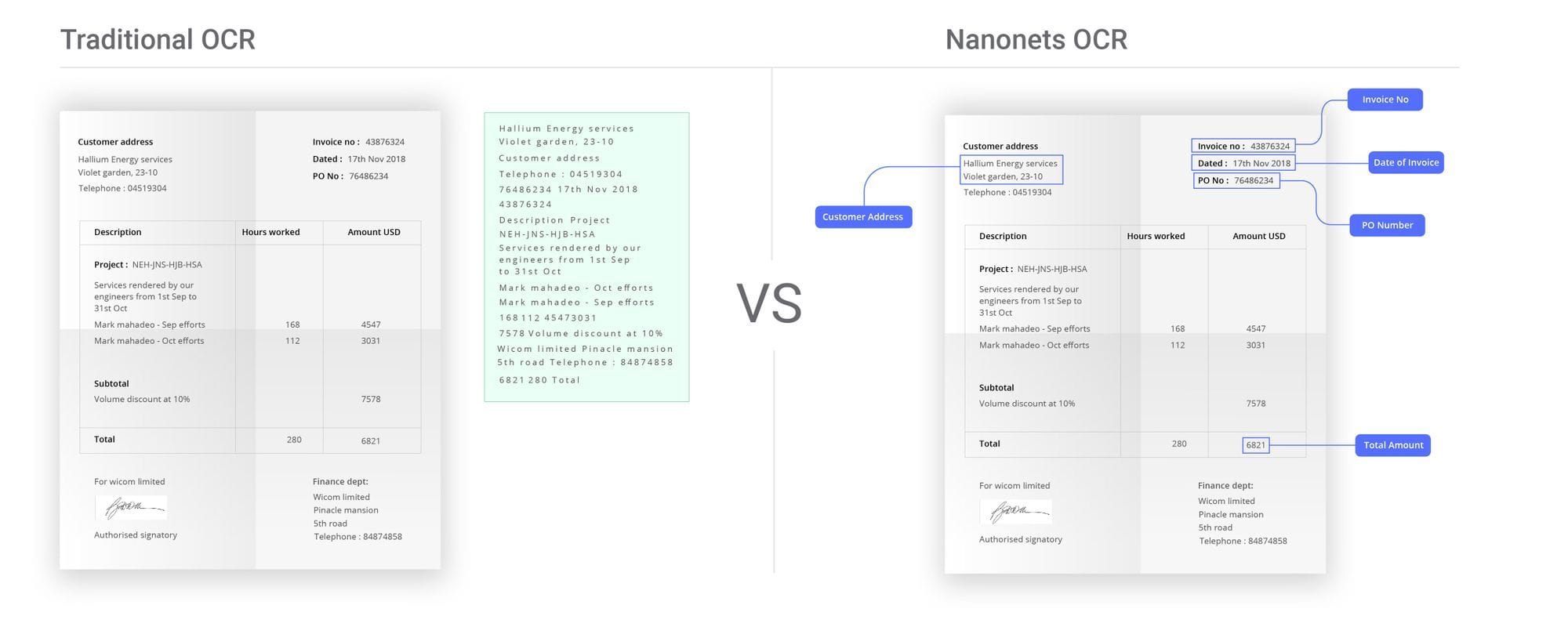
How is unstructured data extraction useful
Unstructured data extraction is essential in today's digital age, streamlining operations and enhancing decision-making across various sectors.
The transformation of raw, unstructured data into structured, easily retrievable formats such as tables enables businesses to access and analyze information previously obscured by its unstructured state. This data restructuring improves usability, removes ambiguity, and facilitates seamless integration into existing data systems.
Significantly, the utility of unstructured data extraction extends beyond mere data organization. In the banking industry, for example, these innovations play a pivotal role in growth strategies, helping to identify trends, optimize customer experiences, and ensure regulatory compliance.
Scientific research also benefits from the precise refinement of data. Tools designed for unstructured data extraction can distill vast amounts of complex information into concise, actionable insights, making them indispensable in the quest for discovery and innovation.
In essence, the extraction of unstructured data is not just about preserving the integrity of information; it's about unlocking potential, fostering growth, and powering progress.
How is unstructured data extraction used in different industries?
Businesses across industries use unstructured data extraction techniques to make sense of their business documents and add more intelligence to their analytics. The figure below shows the advent of unstructured data in different industries.
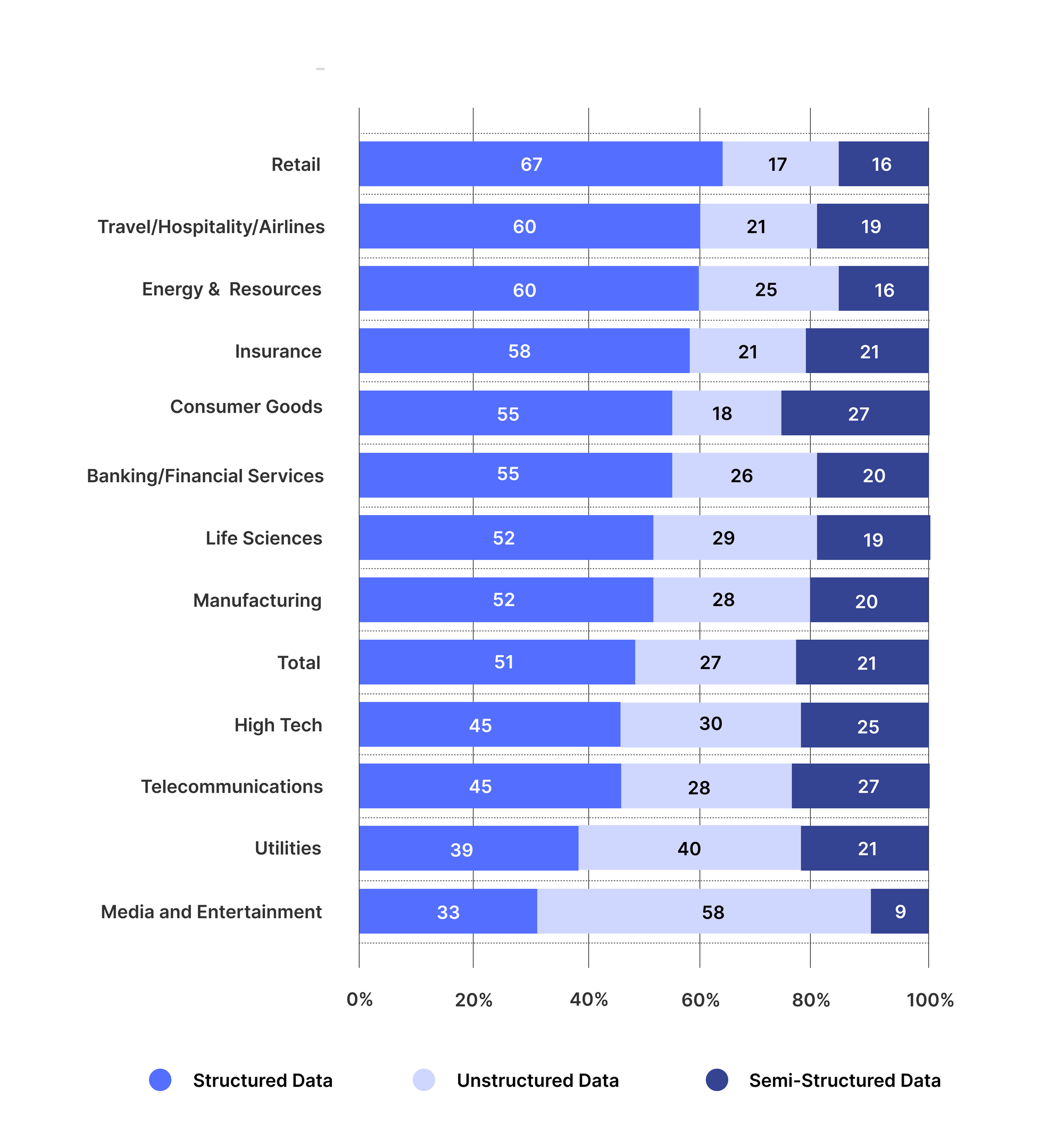
[Source: TCS Study]
Here are some examples of how different industries use intelligent document processing platforms like Nanonets to extract unstructured data and enhance their productivity.
Banks
Banks use IDP platforms to extract insights from unstructured data sources like claims, customer forms, KYC documents, call records, financial reports, and more.
Read more: RPA in Banking and Banking Automation
Insurance
Insurance is a heavily regulated industry. It must perform document and identity verification at every insurance claims process step. Insurance firms use automated document processing platforms to automate claims processes, risk management, and rule-based functions. The insurance claims process contains a lot of unstructured data. Unstructured data extraction using AI-enhanced platforms like Nanonets makes the insurance claims process easy as it allows for selective data extraction from images, PDFs, videos, audio, etc.
Read more: Insurance Automation and RPA in Insurance
Health
Providing exceptional patient experience involves better service, reducing patient wait times, and ensuring staff aren’t overworked. Using the IDP platform to extract insights from unstructured data sources like the voice of customer data, patient surveys, EHRs, customer complaints, regulatory websites, and literature reviews helps Healthcare to ensure a better patient experience.
Read more: Healthcare automation and AI in healthcare
Real Estate
Real estate companies deal with multiple people simultaneously, like customers, builders, tenants, vendors, competitors, and property owners. Using automated document processing software can help real estate institutions create rich profiles of the mentioned stakeholders and streamline the data extraction from unstructured data sources like rent leases, contracts, property valuation papers, etc.
Read More: How to Automate Data Extraction from Contracts?
NLP Techniques for Extracting Information from Unstructured Text
Natural Language Processing (NLP) provides effective techniques to transform unstructured text data into valuable, actionable insights. Using advanced algorithms and computational linguistics, organizations can extract meaning from vast amounts of textual information that would otherwise remain unused.
The following techniques represent the core methodologies that drive modern unstructured data extraction solutions, each offering specific capabilities for different business applications.
1. Sentiment Analysis:
Sentiment analysis, or opinion mining, determines the tone of text data—whether it's positive, negative, or neutral. It can be further categorized into emotion detection, graded analysis, and multilingual analysis, enhancing its precision and applications.
- Emotion Detection: Beyond polarity, detects emotions like frustration, happiness, etc.
- Graded Analysis: Provides a nuanced sentiment analysis, akin to a 5-star rating system.
- Multilingual Analysis: Identifies language in text and applies sentiment analysis.
2. Named Entity Recognition (NER):
NER is a Machine Learning technique classifying identifiers in predefined categories. It can be used for diverse applications, such as training chatbots, medical term identification, and automated categorization of customer issues in customer support.
3. Topic Modeling:
Topic modeling, often implemented using Latent Dirichlet Allocation (LDA), automatically assigns topics to words or phrases in a document. It groups comparable feedback, aiding in the extraction of information from unstructured text based on word/phrase frequency and contextual patterns.
4. Summarization:
NLP summarization aims to reduce document length without altering meaning. Two methods are extractive (selects important words based on frequency) and abstractive (understands meaning for a more accurate summary). Summarization benefits include time savings, increased productivity, and comprehensive coverage of facts.
5. Text Classification:
Text classification, also called categorization or tagging, assigns tags to text based on content. Rule-based, machine-based, and hybrid approaches are employed, utilizing linguistic rules, machine learning, or a combination of both. It serves to analyze unstructured data efficiently.
6. Dependency Graph:
A dependency graph, structured as a directed graph, reveals relationships between words, aiding in the analysis of grammatical structures. Dependency parsing assumes relationships between linguistic units, simplifying the extraction of information from unstructured text by representing dependencies between elements in a system.
Conclusion
Data is the new oil. Enterprises that master unstructured data extraction can unlock the full potential of their data. With Nanonets' AI-OCR capabilities, businesses can leverage AI document processing to automate workflows and extract data from any document with ease.
FAQs
What is the difference between rule-based and AI-driven unstructured data extraction?
Rule-based extraction uses manually created templates and predefined logic, making it effective for structured documents with consistent formats but inflexible when layouts change. It requires constant manual updates and struggles with variations.
AI-driven extraction, by contrast, uses machine learning and NLP to automatically learn patterns from data, handling diverse document layouts without predefined rules. AI solutions are more flexible, scalable, and adaptable, improving over time through training. While rule-based systems work well for repetitive tasks with fixed fields (like standard invoices), AI excels with complex, varied documents like contracts and emails that have inconsistent formats.
How accurate is AI-based unstructured data extraction?
AI-based unstructured data extraction typically achieves 80-95% accuracy in real-world applications, depending on various factors. Modern OCR systems reach 90-95% character recognition accuracy, while advanced NER models achieve F1 scores of 0.90-0.97 for entity extraction from specialized documents.
Accuracy depends heavily on data quality, document complexity, training dataset size, and preprocessing techniques. Clean, high-resolution documents yield better results than poorly scanned or handwritten materials. AI models continuously improve through retraining and fine-tuning, becoming more accurate over time as they process more data. Hybrid approaches that combine multiple AI techniques often deliver the highest accuracy for complex extraction tasks.
Can unstructured data extraction handle multiple languages?
Yes, modern unstructured data extraction systems can effectively handle multiple languages. Advanced OCR engines like Google Vision API and Tesseract support numerous languages, including non-Latin scripts such as Arabic, Chinese, and Hindi.
For text analysis, multilingual NLP models like mBERT and XLM-RoBERTa process content across various languages simultaneously. However, performance varies by language—complex scripts, languages with rich morphology (like Turkish or Finnish), or those lacking sufficient training data present greater challenges.
Language-specific preprocessing, automatic language detection, and custom-trained models help overcome these limitations. While AI-based extraction works across languages, accuracy may still differ between widely-used languages with abundant training data and less common languages with limited resources.
Is unstructured data extraction scalable for large datasets?
Yes, unstructured data extraction can effectively scale to handle large datasets when implemented with the right technologies. Modern AI-based extraction systems use deep learning models (CNNs, RNNs, transformers) that process massive amounts of complex data efficiently.
Scalability is further enhanced through cloud computing platforms like AWS and Google Cloud, which provide elastic resources that grow with your needs. Big data frameworks such as Apache Spark distribute processing across machine clusters, while parallel processing capabilities enable simultaneous data handling.
Organizations can improve performance by implementing batch processing for large volumes, using pre-trained models to reduce computational costs, and adopting incremental learning approaches. With proper infrastructure and optimization techniques, these systems can efficiently process terabytes or even petabytes of unstructured data.