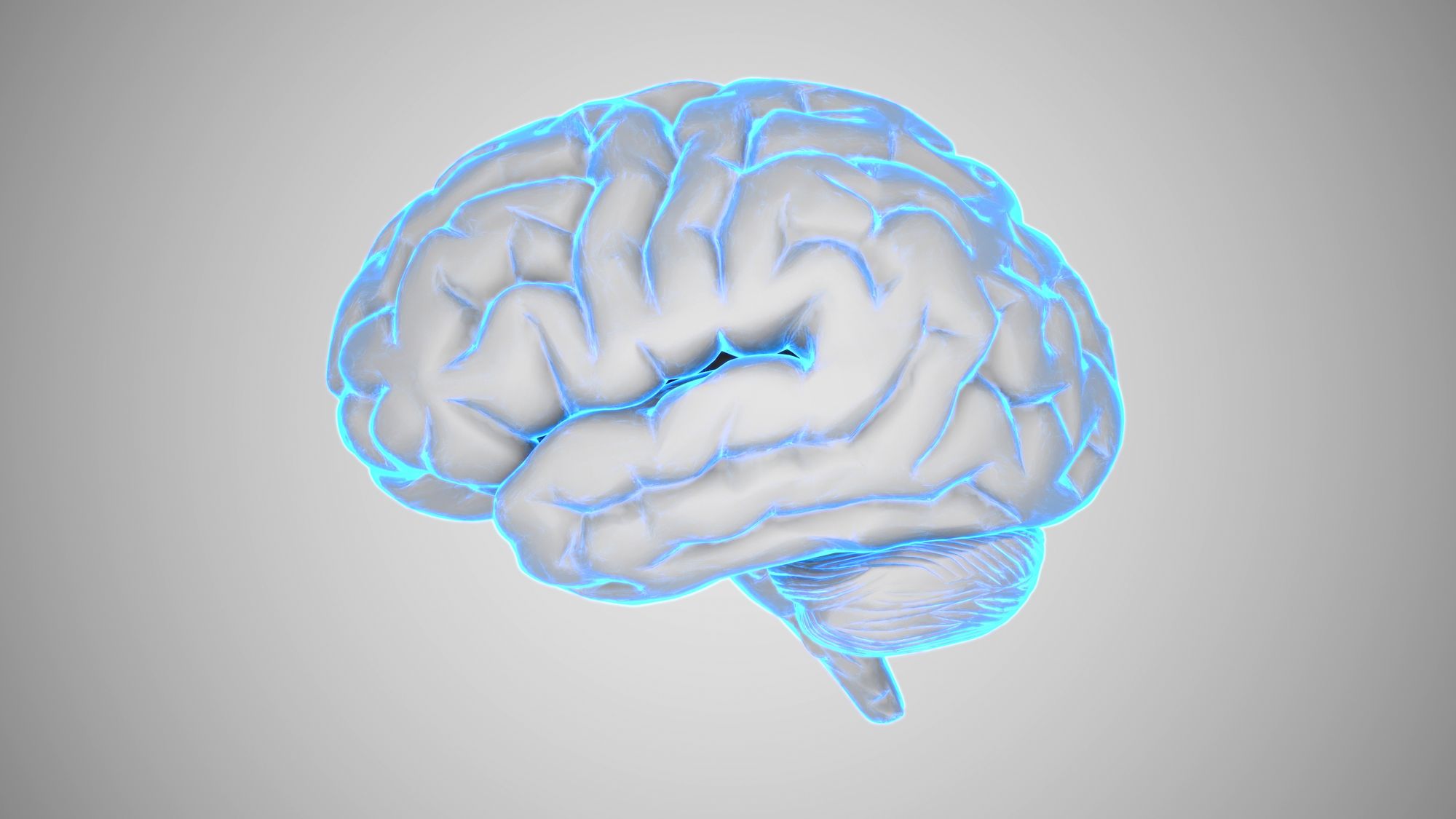
Looking for an automation solution? Look no further!
“As more and more artificial intelligence is entering the world, more and more emotional intelligence must enter into leadership.” -Amit Ray, Famous AI Scientist, Author of Compassionate Artificial Intelligence
The fourth industrial era in which we live is disruptive in that it blends the carbon-based brain with the silicon one. Artificial intelligence is part of our lives already, even if we don’t even realize it – search engines, digital assistants, maps, and navigation, the list is endless. Machines can now “learn” as they work, but this does not, in most cases, exclude humans from the process.
Humans in the Loop or HITL systems allow both forms of intelligence to interact elegantly for their mutual benefit.
Let us learn more about human in the loop AI.
Definition of Human In the Loop
Our machines have come a long way since Paul Ehrlich wrote in 1978 “To err is human, to really foul things up takes a computer”. Today’s Artificial Intelligence tools have progressed so much that the margin of error has decreased considerably. This is important because AI tools are now used in critical applications including flights, life support, and weapons control where mistakes are catastrophic.
That said, AIs, like the human that built them, are not perfect. The predictions made by AI tools are not 100% accurate because machines build their understanding from existent data and patterns. While this is true of human intelligence as well, there is an added element of trial-and-error-based cognition that uses multiple inputs and an added factor of emotional reasoning in human intelligence. This probably makes the human prone to error while the machine, prone to fouling things up.
But jokes aside, AI systems cannot as yet be completely human-free because of this inherent uncertainty of accuracy, and most, if not all, AI tools use some amount of human interaction to course correct or simply monitor. The interaction between man and machine results in a feedback loop that enables periodic course corrections of the AI system to improve performance and enhance autonomy. Thus emerges the formal definition for Human in the Loop.
In effect, human-in-the-loop AI allows humans to provide feedback to the AI model (ML, DL, ANN, etc.) for predictions below a certain level of confidence.
Want to scrape data from PDF documents, convert PDF to XML or automate table extraction? Check out Nanonets' PDF scraper or PDF parser to convert PDFs to database entries!
ML, a popular AI Tool amenable to HITL
Learning is the process in which pre-existing data is used to make future predictions – “a burnt child dreads the fire” is a relatable, if disturbing, example of the learning process. Machine learning, one of the tools of AI, works in much the same way – it learns patterns from existing data and makes predictions based on these patterns. For example, using the images of happy and sad faces from a pre-existing database of emotional faces, an ML tool identifies a new face as happy or sad. The prediction is then validated, and if found correct, moves forward, stashing this new “experience” as another data point. If not, the machine course corrects.
Want to automate repetitive manual tasks? Check our Nanonets workflow-based document processing software. Extract data from invoices, identity cards or any document on autopilot!
Types of HITL in ML
In Human in the Loop Machine Learning, the human participates at many levels.
Creation
The human component starts with creating the algorithm and the algorithm takes off thereon. Much like Tony Stark and his J.A.R.V.I.S.
Training
As described earlier, learning happens with data. When a child does not touch the flame, an adult has probably taught her not to. Human judgment is used to train the model so that in due course the model performs like or outperforms the human in making predictions using patterns.
Labelling data
Machine Learning models need labelled data from which to learn. Some datasets may already have labels, but in the absence of pre-labelled data, humans must label the data that trains the ML algorithm. According to the IDC, 90% of data that is available is dark data, i.e. unstructured/uncategorized data. Labelling may be time-consuming, tedious work. Indeed, data labelling has become a stand-alone job in the field of artificial intelligence and data science. As mundane as it may sound, labelling of the datasets is not always a low-end activity, and specific applications may require domain-specific knowledge. For example, tagging medical data needs knowledge about diseases, conditions, etc. Most datasets used in the health care domain require domain-specific knowledge, like a doctor tagging a lung X-ray as cancerous or not. The tagging of data used to train AI used in flights requires knowledge of aerodynamics and other engineering topics.
Validation
Once an ML model begins predicting using real-world data, the HITL validates the model's predictions and provides feedback about false positives and false negatives to the ML for training. The human in the loop can review the model’s performance and analyze its performance, for tweaking the algorithm or improving the training dataset.
Want to use robotic process automation? Check out Nanonets workflow-based document processing software. No code. No hassle platform.
The importance of human-in-the-loop ML and other AI tools
When there is a dearth of training data
Conventional machine learning and other AI tools require a large dataset to train well and obtain accurate results. In a new field or a field that lacks prior data, ML models are not accurate to start off and take a long time before sufficient data is generated for training. The Human in the loop AI can help in these cases where the human teaches the algorithm, the patterns and the rules without the need for a large dataset to work on. In that context, HITL helps validation of models and allows for training using data that are unstructured, hard to tag, and constantly changing.
When dehumanizing is not an option
There are also specific fields in which the human in the loop of AI is useful, even necessary. One field is health care. While AI can certainly ease diagnosis and even therapeutics, such as robotic surgery, it is unclear whether it can be de-humanized. It is indeed true that AI can help clinicians spend less time on administrative and diagnostic tasks, but debate continues to exist on whether dehumanized AI would undermine the humane dimension of the patient-physician relationship. The general ethical consensus is that the human-in-the-loop is necessary for AI to serve human ends, respect personal identity and promote human interaction.
Where two eyes are safer than the machine vision
HITL is also needed in situations that require utmost precision for safety. An example is the manufacturing of critical parts for vehicles or aeroplanes; while AI tools such as ML are immensely useful for inspections, a human monitor in the group would add to the reliability of the part. Furthermore, with incomplete or biased data, Machine Learning models can themselves become biased. A human in the loop can detect and correct bias in time.
For increased transparency
AI applications can become black boxes in which the processing that converts data to a decision is hidden. This is inconvenient for data-sensitive activities such as finance and banking. This is also a problem for decision-making, regulatory compliance, and disclosure needs that are associated with certain activities. In such cases, the HITL model allows humans to see how the AI tool arrives at a particular outcome with a given set of data. This allows the AI/ML tool to be, in the parlance of thermodynamics, an “open” rather than an “isolated” system.
To empower the AI tool
When a child learns the alphabet, a teacher is required, but as she grows, the role of the teacher becomes guidance rather than teaching eventually, the now-adult can learn by herself without the need for a teacher. Much like that, the human is required to train the system first, and the more the AI tool learns from the human intervention, the better it gets, and the amount of human time in the loop can be reduced, or in some cases, even eliminated. Thus the AI tool benefits from human intelligence through the feedback loop.
In deep learning
Human in the loop deep learning is used in the following scenario:
- Algorithms do not recognize the input data.
- Input data is misinterpreted
- There is indecision on the next task to employ on the data
- To enable human beings to perform certain tasks objectively
- To reduce errors and time delays for human tasks
If you work with invoices, and receipts or worry about ID verification, check out Nanonets online OCR or PDF text extractor to extract text from PDF documents for free. Click below to learn more about Nanonets Enterprise Automation Solution.
Applications of Humans in the Loop
AI and ML systems are omnipresent in the world today. The human in the loop may either be only at the consumption end, or in the operational realm as well. Examples of the former include the use of search engines, digital maps, navigation, etc., in which the human consumer uses an AI system to avail of various services.
Some typical applications in which the HITL is at the stage of the AI/ML operation itself are:
Social Media
The line between the use and abuse of social media applications is fine, and human judgment is essential to moderate content. It is true that AI systems can learn to moderate content over time. But for that, human involvement is essential to help the machine learn to identify text, usernames, images, and videos that may have undesirable elements of interaction.
Health Care Tech
Medical imaging and AI-based recognition of the normal and abnormal features of the image are being extensively developed. Such developments require intervention by subject-matter experts, in order to train the model to look for specific features of the image that point to abnormalities. Even the best-trained models must be backed further by human confirmation because diagnostic and therapeutic services deal with lives, and mistakes are not acceptable. The health care tech applications require intensive data labelling services to augment their training data.
Transportation
Self-driving cars are already nearing practical use, but for further developments, massive amounts of data in the form of images, videos, and sounds must be gathered and annotated by humans. Labelling image data as humans, vehicles, roadblocks, vegetation, animals, road shapes, etc., is of paramount importance for the ML to enable automated driving sans accidents. Enormous human labelling and annotation efforts are needed to realize truly self-driving vehicles in the world.
Defence applications
The futuristic vision for defence organizations is the use of autonomous systems in dangerous missions. Such systems must be able to make human-like decisions under split-second conditions. However, the amounts of data available to train these high-performance AI backends are currently insufficient to enable complete autonomy. Human-free artificial-intelligence systems are also incapable of understanding contextual information in the input and this can result in catastrophic predictions and decisions. Thus, as of now, a human is certainly required in the loop to keep the defence operations under control and human.
Creative applications
Beyond the above “essential” applications, HITL AI systems can have entertainment value as well. The Stanford Human-centered AI initiative designs systems that infuse technology with human interaction to develop new tools for musical and other forms of human creativity. Style-transfer deep artificial neural networks use human intervention to teach machines “styles” of paintings for new AI-creations.
Other fields that benefit from Human in the loop AI systems include sports, games (video and real-life), agriculture, factory automation, and financial activities.
Want to automate repetitive manual tasks? Save Time, Effort & Money while enhancing efficiency!
Take away
We are still a long way, if at all possible, for robots to rise and take over the world. Humans are still required in the loop of artificial intelligence. The broader approach to AI is not the design of a perfect machine – which is exceedingly difficult, if not impossible, but the design of collaborative systems that combine the subtlety of human reasoning and the power of intelligent automation.
Nanonets online OCR & OCR API have many interesting use cases that could optimize your business performance, save costs and boost growth. Find out how Nanonets' use cases can apply to your product.