In 2023, the U.S. loan market hit $12 trillion. From buying homes to expanding businesses, borrowers flood lenders with applications, yet the traditional loan underwriting process remains slow and manual.
Enter AI-powered automation – a revolutionizing process, enabling faster, smarter lending decisions.
With automated loan underwriting, lenders can cut through the paperwork, reduce approval processing times, and make smarter, data-driven decisions. Underwriters can analyze a borrower’s credit history, income data, and employment status instantly — compared to the days or weeks it takes through traditional methods.
In this article, we’ll dive into how AI can transform the loan underwriting process and solve some of your biggest challenges. You will also learn how to set up an underwriting automation workflow.
What’s wrong with manual loan underwriting?
For most loan underwriters, the process is lengthy, time-consuming, and full of manual tasks.
Traditional underwriting usually takes 30 to 45 days.
This time frame can increase further when dealing with complex mortgage loans. Here's why:
- Loan officers gather extensive documentation from applicants - credit reports, bank statements, tax returns, pay stubs, employment verification, etc.
- Verifying data across different sources and evaluating a borrower’s financial standing takes several days or weeks.
- Human errors affect many underwriting decisions, resulting in inconsistencies and delays. Data entry, calculations, and approvals inherently increase the duration.
- Underwriting requires back-and-forth communication between the lender, applicant, and other third parties (like employers or credit agencies). Delays in receiving responses or additional documents further prolong the process.
- Manual loan underwriting also leaves room for subjectivity. In fact, many loan rejections are attributed to human bias or fatigue. Ensuring compliance with regulatory requirements adds another layer of complexity and effort.
This conventional approach slows down approvals and ties up underwriters in repetitive tasks, limiting their capacity to focus on strategic decision-making.
Enter automated loan underwriting
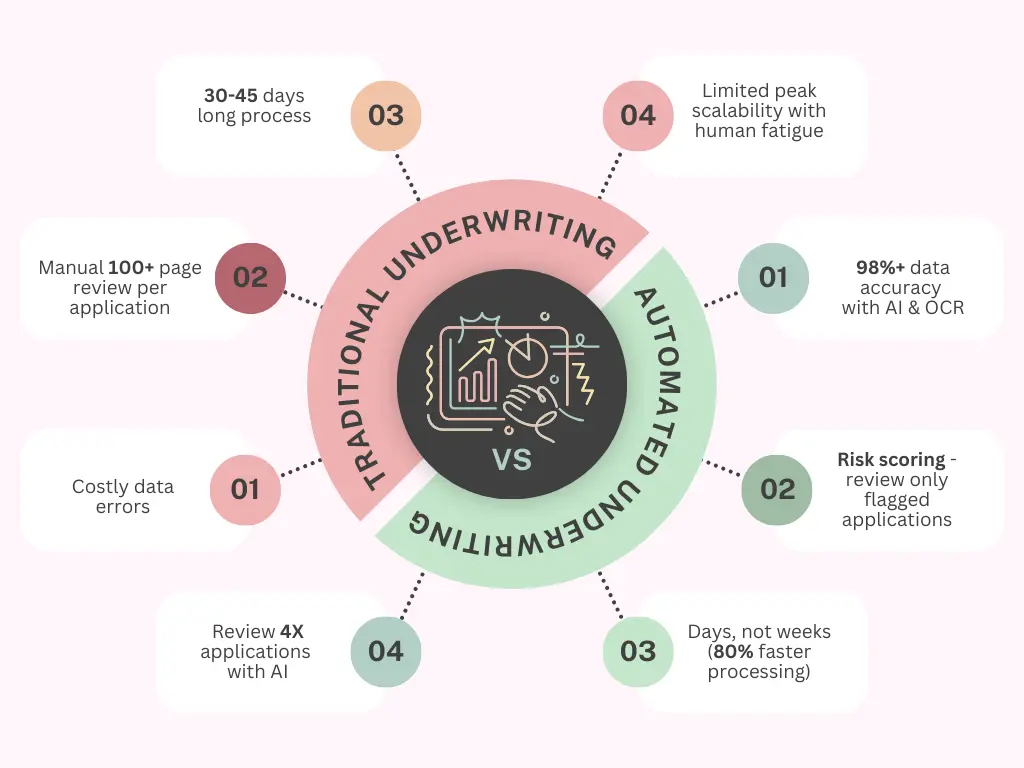
Loan underwriting automation uses artificial intelligence (AI) and machine learning (ML) to streamline the traditionally manual process of assessing borrowers' financial standing, collecting documents, and making loan approval decisions.
AI can reduce application processing times by up to 80% by automating document collection, eligibility verification, and risk analysis.
Automated systems offer unparalleled scalability. By handling volume increases of up to 400% without additional staffing, these AI-powered tools allow lenders to manage demand fluctuations efficiently.
Key aspects of loan underwriting automation
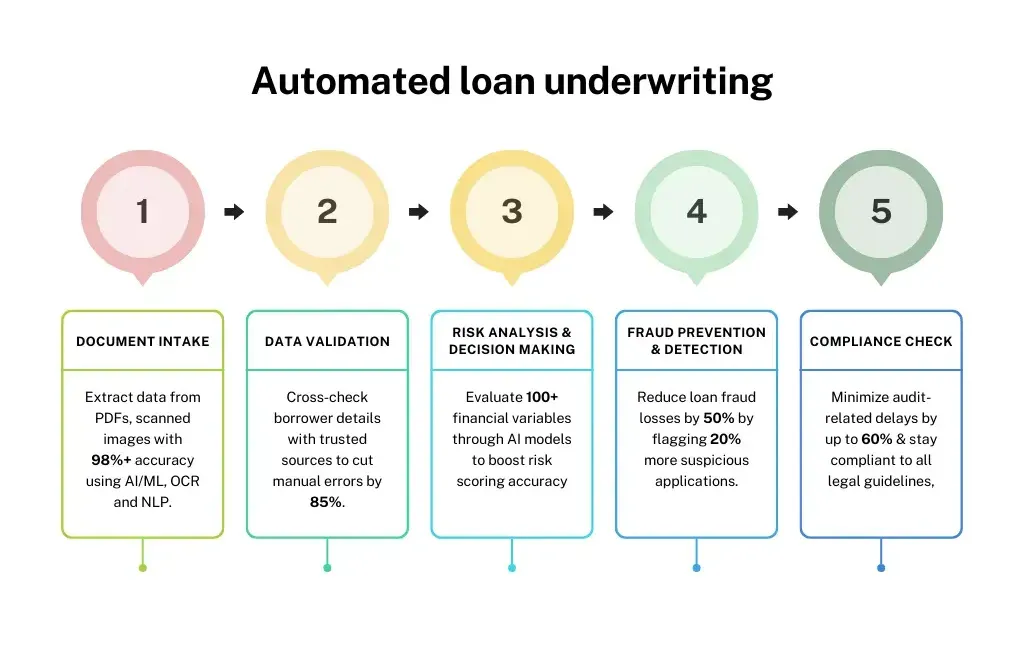
Data extraction
Automated data extraction leverages technologies like Optical Character Recognition (OCR) and Natural Language Processing (NLP) to extract and process data from various documents, such as loan applications, bank statements, and credit reports.
- Advanced OCR tools extract data from structured and unstructured documents, making processing different file types, such as PDFs, scanned images, and application forms easier.
- Data digitization eases access to information, thus addressing the issue that 93% of businesses face in locating important borrower information.
NLP enhances data extraction by understanding the context, meaning, and sentiment behind text.
- Keyword extraction automatically highlights critical information like income, liabilities, and risk factors, allowing underwriters to assess essential data in loan applications quickly.
- Named entity recognition (NER) identifies key entities such as names, financial figures, and dates, speeding up the verification of applicant details and improving creditworthiness assessments.
- Text summarization condenses lengthy documents (e.g., tax returns and financial statements) into concise summaries, enabling underwriters to review essential information faster and make quicker decisions.
– Jeffrey Zhou, CEO & Founder, Fig Loans
Data validation
Inaccurate data affects 88% of companies and costs an average of 12% of revenue. Ensuring the accuracy of financial and personal data is crucial to loan underwriting, reducing risk, and making sound lending decisions.
Here’s how automation enhances data validation in underwriting:
- Automated data cross-checks verify extracted information against trusted sources like credit bureaus, tax records, and banking institutions. Automating data validation and risk evaluation reduces decision-making times by 60-70%, speeding up the loan approval process.
- Error detection flags inconsistencies such as mismatched income statements or credit scores, allowing underwriters to address them quickly.
- Fraud prevention tools analyze documents for signs of forgery or tampering (e.g., fake bank statements), reducing fraud risk losses by up to 50%. These tools also validate that all required documents meet compliance standards before proceeding to loan approval, reducing the risk of penalties during audits.
Automation ensures compliance with legal and financial guidelines, helping lenders avoid penalties.
Risk analysis and decision-making
AI and ML tools help underwriters assess an applicant’s financial health by analyzing factors such as credit history, income stability, and spending patterns.
- Comprehensive risk scoring generates accurate risk scores, helping underwriters make informed decisions quickly and consistently.
- Automated algorithms evaluate multiple variables such as credit scores, income levels, and financial history, minimizing bias and improving decision-making accuracy.
- Fraud detection and predictive modeling allow underwriters to forecast risks, reducing default rates by 20%.
Compliance checks
Finally, compliance checks are integrated into the underwriting process to ensure adherence to regulatory standards and minimize the risk of violations. Automated systems validate that all required documents meet compliance standards before obtaining loan approvals.
Continuous training of our data extraction tools and regular audits keep us aligned with changing regulations, while robust validation safeguards against errors, protecting client data and trust."
– Andrew Franks, Co-Founder, Claimsline
How to solve challenges in loan underwriting with AI
Find below some common challenges in the loan underwriting process and tips on handling them using automation and AI:
1. Manual document handling
Manually extracting relevant data from varied formats is time-consuming and error-prone.
In one instance, a misplaced decimal point in the income field caused by misreading a blurry document led to an inflated income figure, resulting in a wrongful loan rejection. Misreading or incorrectly transcribing this data delays the process and risks making inaccurate lending decisions.
AI tools can handle diverse document formats, ensuring data capture with 98%+ accuracy and significantly reducing manual errors. This speeds up the underwriting process while minimizing the risk of incorrect approvals or rejections.
2. Inconsistent data quality
Inconsistent or incomplete data requires manual intervention and slows the entire approval process.
The system can also send automated reminders to borrowers, prompting them to provide required documents before the application progresses. This reduces the need for manual follow-ups and helps catch issues early.
3. Long approval cycle
However, the traditional review process involves reviewing hundreds of pages of financial statements and causes many delays. Each stage—document collection, data entry, and verification—takes days or weeks, putting the time-sensitive purchase at risk and frustrating the borrower.
Automation eliminates the need for manual review of each document. It only requires human oversight for flagged documents or at the final review stage, reducing the entire process from weeks to days.
4. High operational costs and limited scalability
The added labor costs are high, and errors—such as incorrectly entered credit scores or loan amounts—slip through, leading to rejected applications and expensive rework. This reliance on human resources increases operational costs and the risk of costly mistakes.
Scaling the process is difficult because adding staff quickly is not viable. It is challenging to meet increased demand without sacrificing quality.
These systems achieve accuracy rates far exceeding manual methods, processing thousands of underwriting documents with a minimal margin of error. With AI, businesses can scale their operations without needing additional staff for manual tasks.
Automated workflows can handle a much higher volume of applications without adding staff or reducing accuracy, ensuring the institution can meet demand during peak periods without compromising service quality.
5. Inconsistent decision-making
These systems ensure decisions are made based on uniform parameters, leading to fairer, more reliable outcomes and improving borrower trust.
6. Inefficient risk assessment
As a result, a high-risk loan is approved, leading to future losses for the lender. Manually assessing large datasets increases the likelihood of missing critical details that could indicate a potential risk.
These models provide underwriters with detailed insights, ensuring better risk assessments and reducing the chances of overlooking critical data.
7. Difficulty in fraud detection
Manually detecting fraudulent documents or applications is inefficient and error-prone, allowing fraudulent activity to go unnoticed until it is too late.
In 2020, the Federal Trade Commission reported 204,967 loan fraud cases in the U.S. alone.
These systems cross-check information across multiple applications and databases, spotting patterns linked to fraudulent activity and significantly reducing risk.
Setting up an automated loan underwriting process
Here’s how you can set up and automate your underwriting workflow using Nanonets for loan underwriting:
Step 1: Create an account on Nanonets.
You can sign up for free at app.nanonets.com
Step 2: Choose a pre-built or zero-shot model for extraction
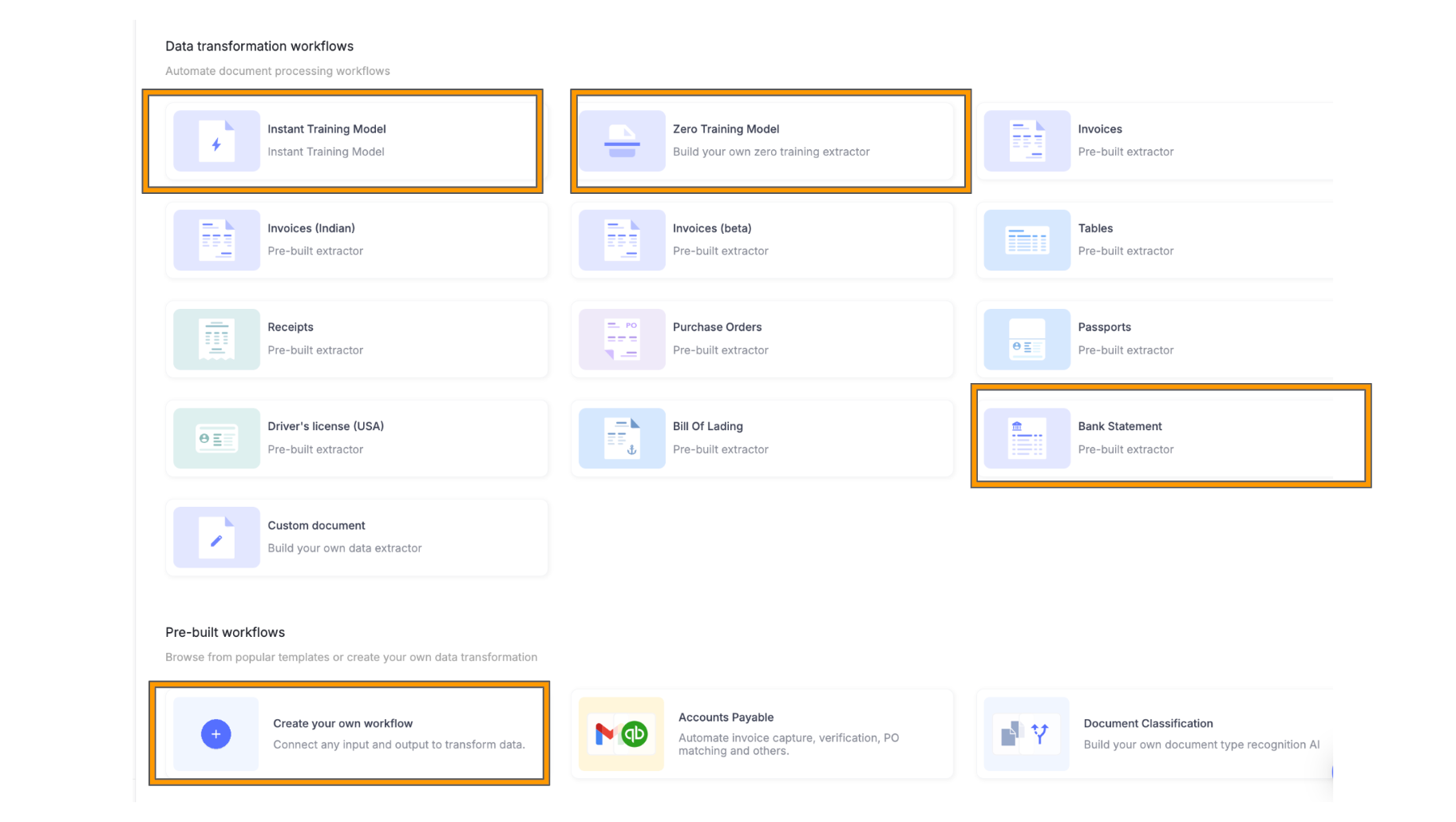
Zero-training models use AI to extract data and do not require any initial training.
You can also use a pre-built extractor for standard documents like bank statements. This will simplify your setup process.
If you prefer to create a custom workflow, select “Create Your Own Workflow” and upload all your underwriting documents, such as applications, W2 forms, financial statements, loan application forms, and claims.
Step 3: Configure import and export settings
While your documents are being processed, configure your import and export settings based on their source.
Nanonets supports over 30 sources, including systems like Dropbox, Google Drive, and email platforms, allowing you to effectively tailor your underwriting automation workflow.
For exporting, you can integrate with any of the tools, like an SQL database, directly or set up an API integration for a third-party tool not on the list.
Step 4: Review extracted fields
Review the extracted fields for accuracy once the workflow is established and the documents are processed. Approve the documents when everything is ready.
Ensure that the extracted data aligns with your underwriting criteria and requirements.
Step 5: Customize your workflow
Enhance your workflows by enriching your extracted data with:
- Data actions: Include steps like lookups, calculations, checkbox detectors, currency detection, date format changes, adding Python code.
- Rule-based approvals: Set up multiple reviewers and validation rules to ensure thorough oversight and compliance - for example, set up a flag for overdraft bank statements. This can drastically reduce manual work by 70-80%.
Step 6: Set up notifications and alerts
Keep your team informed with timely reminders via Slack and Email notifications anytime a new document is uploaded or ready for review.
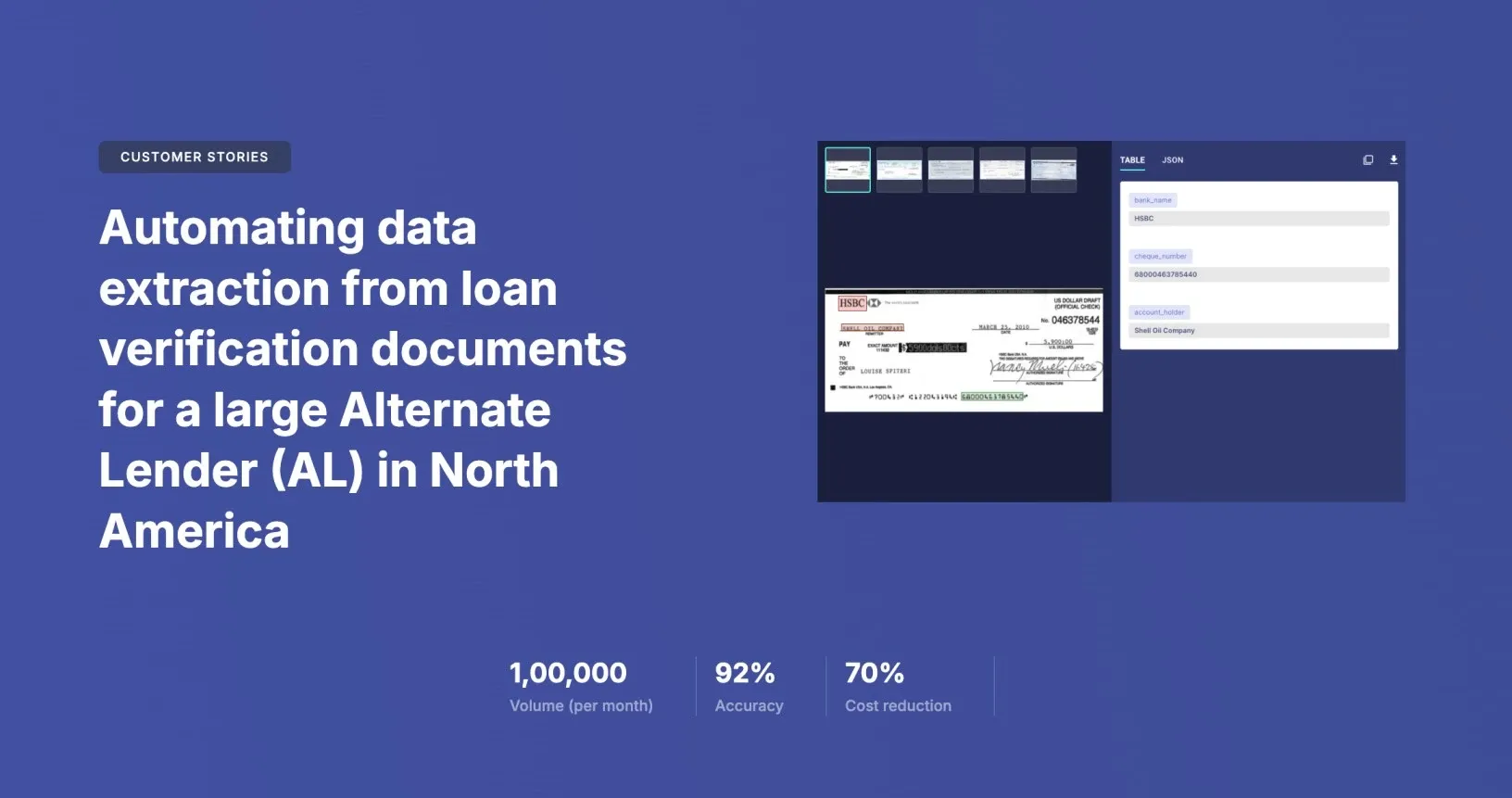
Advanced use cases for document automation in underwriting
Document automation goes beyond simple data extraction, transforming the loan underwriting process by automating complex workflows, reducing obstacles, and improving decision-making accuracy.
The impact extends to multiple underwriting stages, allowing lenders to focus on strategic tasks rather than manual data entry. Below are some specialized use cases for leveraging document automation in loan underwriting:
Automated income verification
Income verification often involves analyzing multiple types of income documentation, such as payslips, W-2s, tax returns, personal finance statements, Profit and Loss statements, and more.
Document automation systems extract key data fields like gross income, deductions, and net earnings in a structured format, flagging inconsistencies like income gaps or unexpected cash flow fluctuations.
The automation tool can further compare extracted data against bank statement transactions to detect potential misreporting or hidden sources of income.
Credit history deep dive
Rather than merely generating a credit score, automation can perform a granular analysis of the borrower’s credit behavior, such as identifying trends in credit utilization, detecting sudden spikes in credit usage, and evaluating payment patterns over time.
It can also integrate non-traditional credit data, like rental or utility payments, to offer a broader perspective on creditworthiness.
Fraud detection
Document automation tools use ML models to detect anomalies and suspicious activity patterns across documents submitted by the borrower. For example, the system can flag this discrepancy if the reported income in a paystub does not match a submitted tax return.
Automation tools can also cross-reference data from public databases and social media profiles to validate the authenticity of submitted information and identify potential identity theft or document tampering.
Automated document categorization and routing
A significant challenge in underwriting is managing the vast array of documents involved—loan applications, identity proofs, income statements, and more. Automated systems can intelligently classify and route these documents based on predefined categories.
For instance, a borrower’s credit report is automatically routed to the credit analysis team, while appraisal documents are sent to the valuation team. This minimizes the chances of misplacing critical files and speeds up review times.
Customer communication and document request automation
Automation tools can handle borrower communications by sending automated requests for missing documents, status updates, or clarification on inconsistencies.
For example, if a borrower fails to submit an updated tax return, the system can automatically trigger a reminder email, reducing the need for manual follow-ups and improving response times.