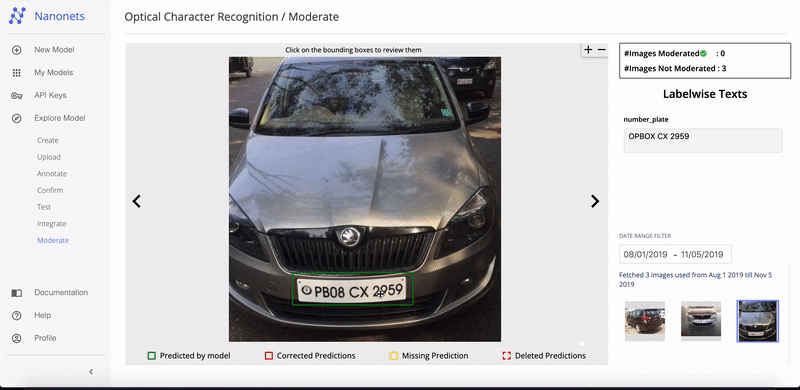
Introduction
This article will take you through what digital transformations are, what drives it, how to aid successful digital transformations, how AI and deep learning can help, the challenges you might face in implementation and how to work around them. We will also talk about what the current pace of technological growth means for the future of work and what we can do about the paranoia that goes along with increasing automation. While talking about singularity or Skynet taking over is not the point of this blog, it would be a little apathetic to not acknowledge the risks that come with acceleration in technological advancement.
Digital transformation
As technology progresses, so do our workflows, the structure of our institutions and organisations and the tools that aid us in performing the tasks required. This requires an organisation to develop new competencies, like being more customer-centric, innovative, streamlined, efficient, being able to process the ever growing volume of information and tap into these new mediums of revenue. Building a digital transformation strategy for a business can be laden with obstacles and requires us to think about a variety of things including collaborations, culture, ecosystems, empowerment, etc and having a clear roadmap to make such a transition is a difficult thing to formulate.
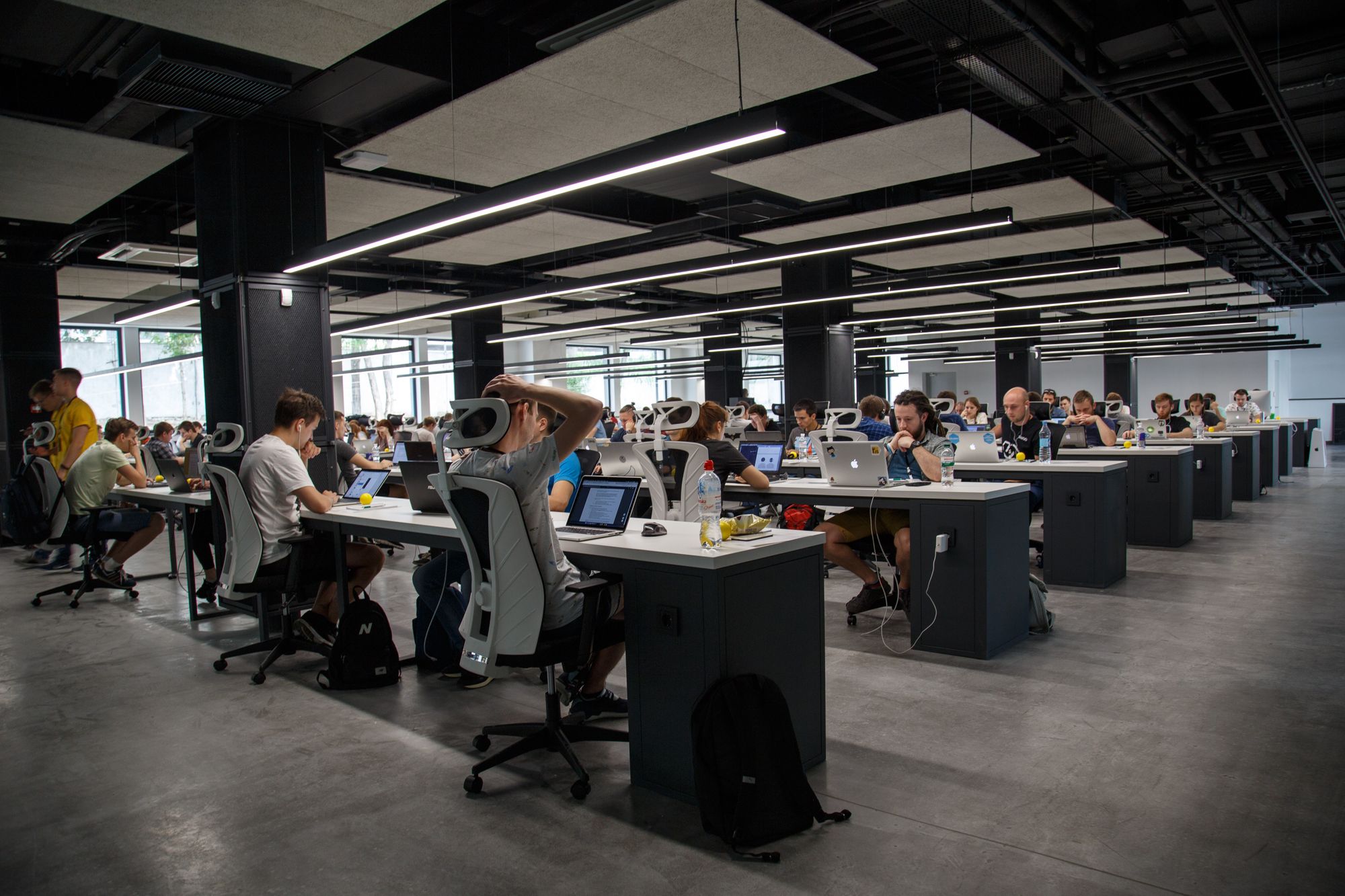
According to this McKinsey article, less than 30% of digital transformations are successful. The number is even lower for digitally savvy organisations like media and telecom companies - less than 26%. The numbers fall even lower in traditional industries like those in oil and gas, infrastructure and pharmaceuticals with a success rate somewhere between 4 and 11%.
Understanding why you need a transformation, and formulating a comprehensive strategy to achieve clearly defined goals needs good leadership who have a good understanding of several facets of the business world.
Ensuring a successful transformation
Ensuring a successful transformation requires an organisation to perform on several levels, requires its people to be more engaged, productive and eager to find innovative solutions to new or persisting problems.
Some factors that can decide whether your organisation's efforts are successful include
- Good leadership, visible engagement of the higher management and effective channeling of workforce to maximize output.
- A cohesive strategy to tie different departments like sales, marketing, supply chain, business development and technology together.
- Clear communication of vision, goals and responsibilities between colleagues, teams, departments and higher management.
- Innovation on technological, cultural and organisational fronts.
- Automation to make easier workflows, enhance productivity, increase efficiency and revenues.
- Connectivity between various departments, customers and suppliers, and prompt response systems in place.
- Willingness of employees as well as convenience for customers to adapt to a changing technological landscape.
- Decision making that is driven by strong insights drawn from data spread over time and capturing multiple landscapes and scenarios
- Developing talent and skills required to sustain the transformation in the future.
- Upgrading day-to-day tools to enable better digitization of information and better user experience.
You can find a much better resource here if you intend to dive deep into digital transformations, how they can be implemented, what to be cautious about and the key factors that make a transformation successful. Here's an article that runs the reader through how to ride the wave of regulatory changes related to privacy and money laundering with the help of AI and deep learning.
Information as an enabler
Over time, we have seen a shift towards the platform economy that is driven by cutting edge technologies like artificial intelligence, big data analytics, cloud computing and internet of things. Staying relevant in an era of companies like Airbnb, Uber, Amazon, Google, Salesforce and Facebook requires us to adopt a data centred and information driven approach. Understanding the power of information and how it can aid digital transformation is key.
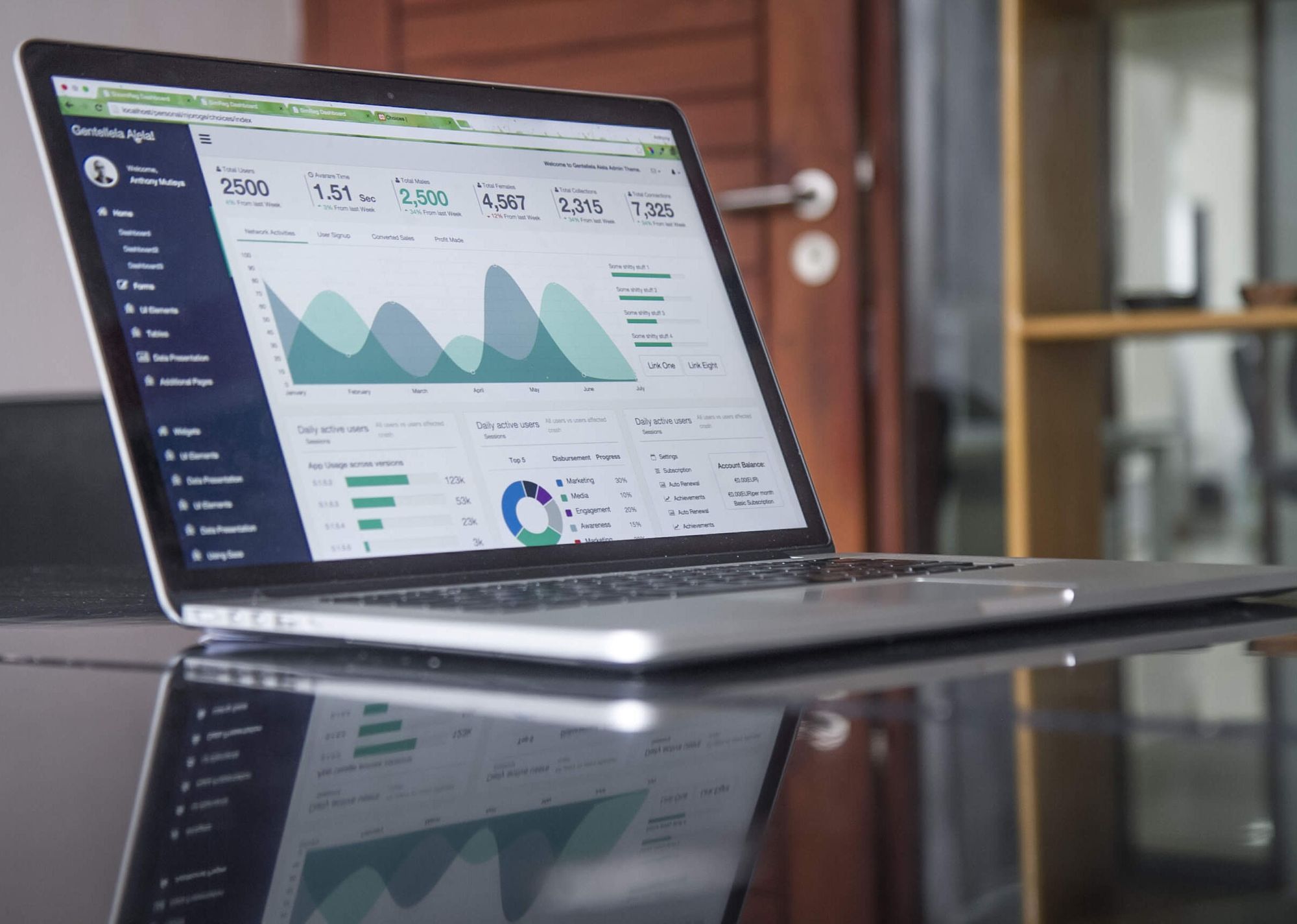
- The what - There is a constant flow of information in several mediums - images, articles, reports, research papers, printed documents, digital documents, etc. A company, depending on what they do might have to deal with a few or many of these mediums. Often, there is all this information companies are collecting from their customers and clients that they do not know how to use and generate value out of.
- The why - Determining your goals for a digital transformation beforehand can go a long way in building a robust strategy based on strong metrics and KPIs. Is your end goal to increase sales and revenues, is it to drive a cultural shift towards newer technologies or maybe to promote better customer experience? Knowing what you want at the end can help the data scientists and engineers in designing solutions in a manner that aligns with the company's broader vision.
- The how - Several things need to be discussed like the feasibility of the product with the engineering and infrastructure managers, training data, defining KPIs, algorithms choice/design, implementation and integration, testing and deployment. A convenient workflow that automates digitization of information, makes reviewing and error correction easier, makes it accessible to anyone without much knowledge of data science or software development can go a long way in driving a successful transformation.
Aiding transformation with AI
Machine learning and artificial intelligence can help companies automate several tedious, time consuming and repetitive tasks and free up time and valuable human resources for more important tasks.
Take the example of a supply chain management system which helps businesses manage inventories, payments, sales, distribution, etc. In a traditional software, the data about receipts and goods bought and sold would need to be entered manually. Multiple people would be employed solely to review receipts and invoices.
The benefits that digitizing these invoices and receipts can cause are endless if the now digital information is processed using machine learning based tools. You can learn more about digitization here. Image quality, information extraction, information structuring, error correction, data entry, data storage - all of these steps that usually require multiple people spending hours and hours to get the job done, can be semi-automated, reducing the number of people required to the same amount of work, that too in a fraction of the time it required before.
Digitizing information
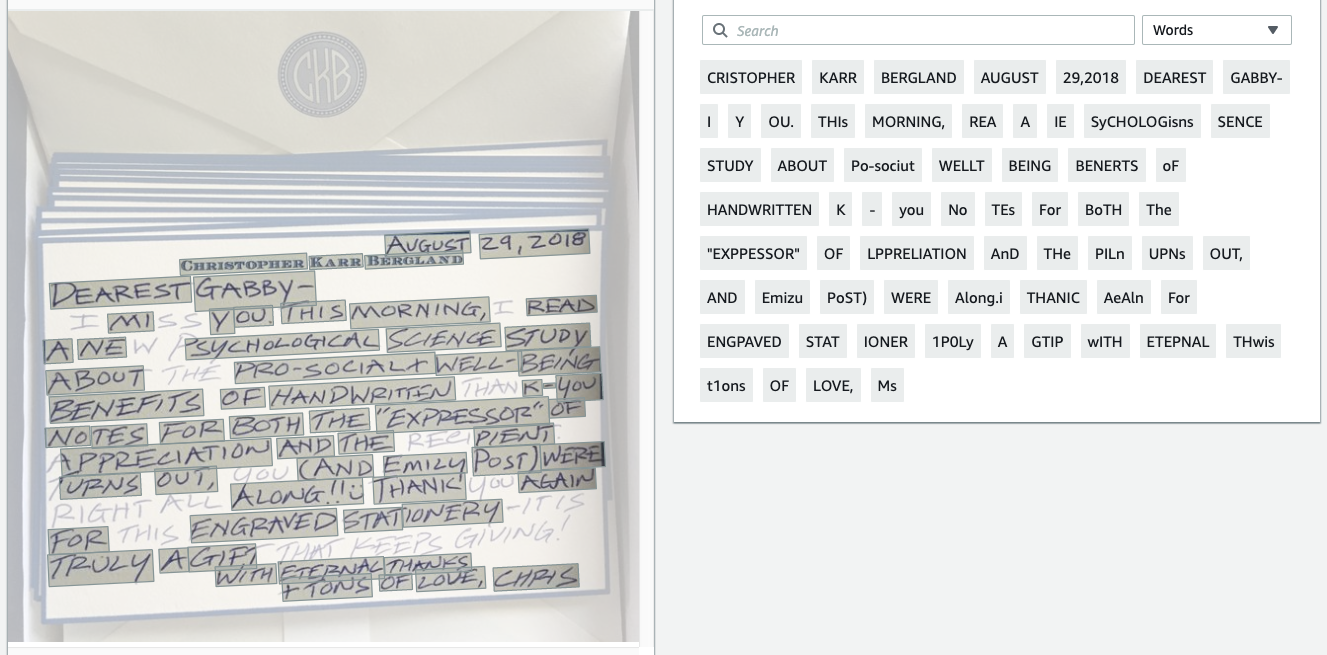
Deep learning approaches have seen advancement in the particular problem of reading the text and extracting structured and unstructured information from images. To make this digitization work for you, there are several things you need to be sure of. Some of them are mentioned below.
- Processing data - The data gathered has to be processed appropriately so our algorithms perform well. This involves cleaning our dataset, balancing classes, making sure of the quality of our data, etc. Noisy and blurry images can severely hurt your predictions. Insights drawn from messy and inconsistent data can lead to disastrous decisions.
- Ensuring accuracy - While research in machine learning constantly strives for generalizability in their learning algorithms, achieving a truly general intelligence is a far fetched goal for any company trying to leverage the technology for their benefit. Different use cases can afford different error rates which in turn decides how your machine learning goals are defined.
- Handling storage and retrieval - A platform or a software that is processing information needs to have robust backend integration with company's databases and a robust infrastructure built on strong big data technologies to be able to perform operations at scale with low latency, minimal downtime and high fault tolerance.
- Easy accessibility - A workflow that is evident, easy to understand, easy to work with and requires little pre-requisite knowledge on the user's part can help a transformation go smoothly. Allowing humans in the loop can help process information and review it faster and with less errors. At scale, this helps increase productivity in the organisation.
Implementation challenges
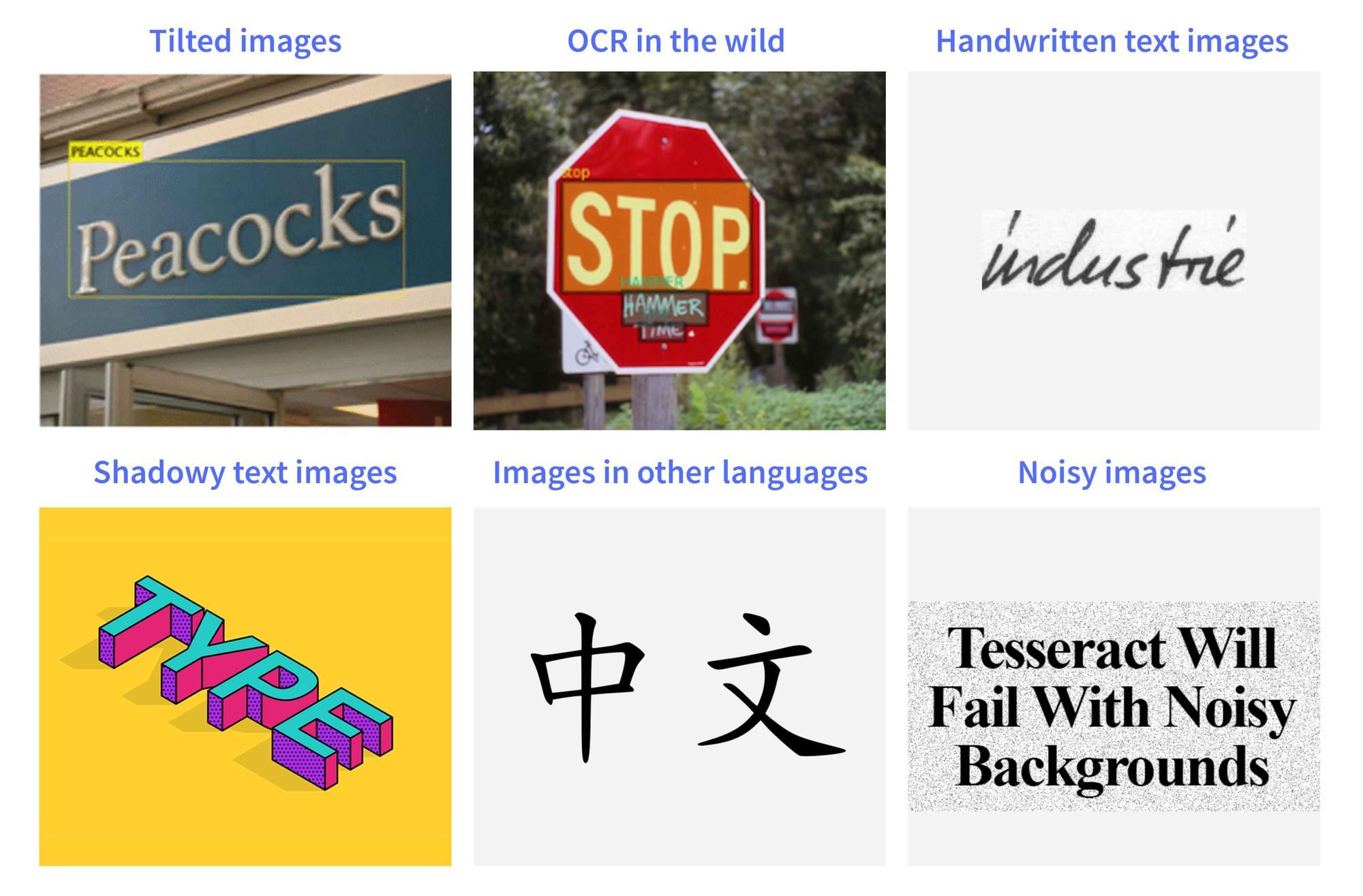
Automating processes using deep learning comes with a lot of obstacles and an end to end, completely automated pipeline for tasks relating to digitization, entry and reviewing is very difficult to achieve and often an impractical goal to have. Some of the problems you might be faced with trying to apply deep learning and artificial intelligence to aid a digital transformation driven by digitization efforts are -
- Lack of training data - Several kinds of documents to be analysed means that you need models that can work with several templates, languages, fonts, font sizes, colors, backgrounds, orientations, etc. A one model fits all solution is almost never possible for a task as diverse and it requires careful collection of data for each model to be able to execute effective machine learning models.
- Image quality - Very often, the images of documents or receipts and invoices your employees are dealing with are taken with different phones equipped with cameras that quality-wise fall in a diverse range, all performing in different light conditions, in various backgrounds, clicked in different orientations and all with different capacities to effectively deal with noise, blur, etc. Dealing with these issues with effective and robust algorithms is key, but can a daunting task if you deal with several documents of various kinds on a daily basis.
- Character accuracy vs sequence accuracy - Automating digitization and reviewing tasks requires the accuracies to be extremely high to avoid inconsistent data storage. While current OCR techniques can provide an acceptable character accuracy, the same models when measured for sequence accuracy often perform far below our needs and expectations. While having models that overfit on your data for niche use-cases might work, at scale, involving humans in the review and correction loop is the most prudent and efficient way to go.
How to make it work
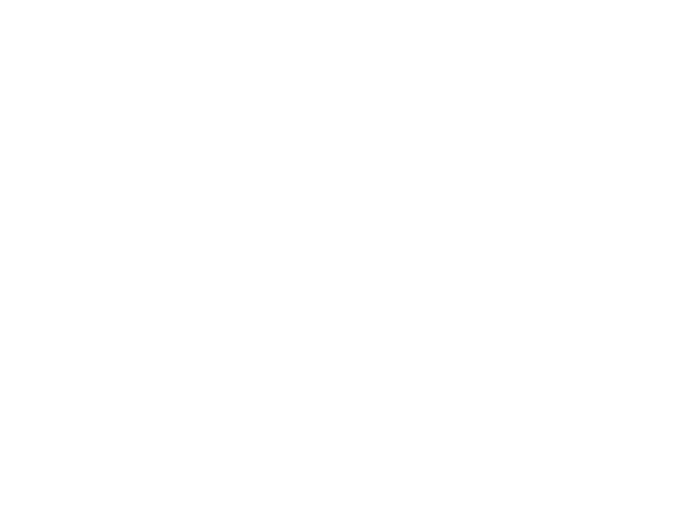
Deep Learning methods have been able to aid the OCR technology to make digitization easier and automation possible. Architectures like spatial transformer networks, attention mechanisms, recurrent convolutional networks (an overview and a tutorial on the tech can be found here) and graph convolutional networks have helped us achieve high accuracies delivered in less time, making it possible for processing documents, receipts, invoices, etc. with minimal errors and paving our path towards automation. Here are a few ways how deep learning makes it possible -
- Automating information extraction - With machine learning, you don't have to spend time going through images of several documents, reading information about customers, data about transactions, prices of products bought or sold, etc. when a machine can handle it for you. OCR allows us to automatically extract information in images and convert it to machine readable text that can be put into the right structure.
- Human-in-the-loop information review - Once the information is extracted, it can be reviewed by a reviewer to make sure the spellings are correct, the information is entered in the right fields, etc. This is almost a 90% reduction in time when compared to processes that require us to read the data and enter it into a software manually. We will talk more about why 100% automation is not feasible in the next section of the article.
- Improving accuracy and performance - Even if the process is completely done by humans, businesses are still often faced with bad data entry practices by their employees leading to inaccurate information stored. This can be remedied with well trained models that can perform at least as well as humans in a much shorter time. Continuous learning and integration in a production environment also helps the models get better over time.
Have a data extraction problem in mind? Head over to Nanonets and start building models for free!
AI, automation and the future of work
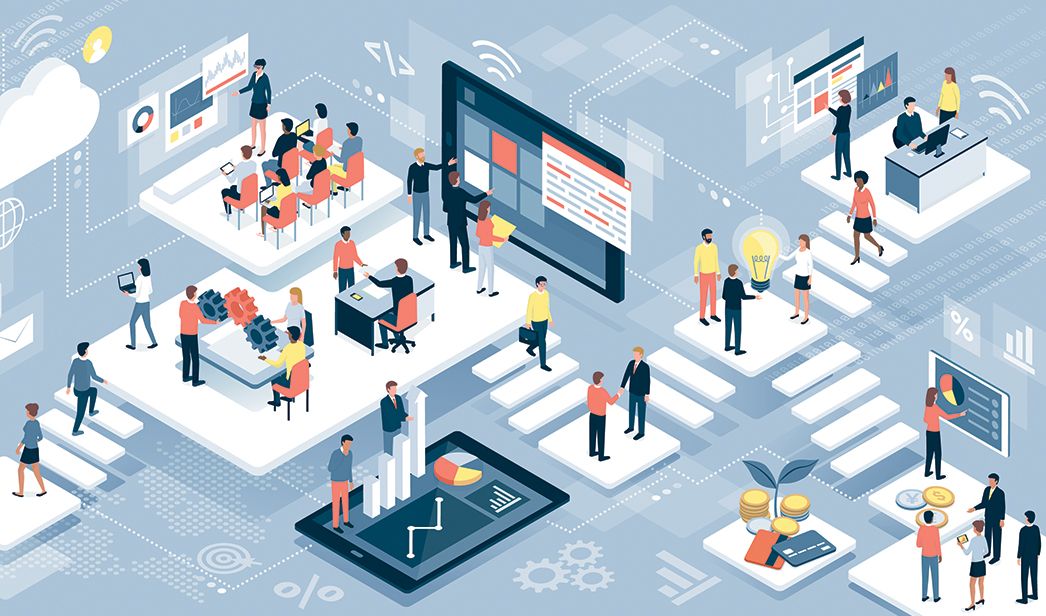
By merging existing deep learning methods with optical character recognition technology, companies and individuals have been able to automate the process of digitizing documents and enabled easier manual data entry (or automated data entry) procedures, better logging and storage, lower errors and better response times. But automation hasn't always been taken in the most positive light by a big chunk of population.
Creating opportunities or taking them away?
As mentioned in this article,
"Bureau of Labor Statistics data show that there’s an unbalanced gender distribution among the most common jobs in the U.S. today. Jobs such as elementary and middle school teachers, registered nurses, and secretaries and administrative assistants each comprise at least 80% women; while jobs such as truck drivers and construction laborers employ more than 90% men."
The author points out that under-representation of women can be tackled with organisations being cautious about using gender-biased tools for recruitment purposes and that having more women in a diverse set of roles can make finding these biases and acting upon them easier.
The paranoia that technology will take our jobs away is real, as is demonstrated in a 2017 survey mentioned in this article about the episode "Metalhead" from the show Black Mirror. According to the survey, 26% of the people interviewed believed they would lose their jobs to advancements in technology in the next 20 years.
According to this article,
"about half of the activities (not jobs) carried out by workers could be automated. Our analysis of more than 2000 work activities across more than 800 occupations shows that certain categories of activities are more easily automatable than others. They include physical activities in highly predictable and structured environments, as well as data collection and data processing."
While this is definitely a cause for worry, the numbers mentioned above are a little misleading. Accelerating progress in AI and automation is creating opportunities for businesses, the economy, and society.
The problem is a two faced one - the first part where artificial intelligence technologies are proliferated in a way that doesn't replace humans but aids them in their work while also creating new markets, opportunities and jobs that did not exist before. The other side of the puzzle is to show people how it is possible, through numbers and experiences, for technology to make their lives better, jobs easier, lives more satisfying and fulfilling. Accelerating progress in AI and automation is creating opportunities for businesses, the economy, and society.
Machines as helpers, not threats
While the article mentioned above mentions -
"...Nearly all occupations will be affected by automation, but only about 5 percent of occupations could be fully automated by currently demonstrated technologies."
It also says this -
"...Many other new occupations that we cannot currently imagine will also emerge and may account for as much as 10 percent of jobs created by 2030."
Partial automation will become much more prevalent in the future like at Amazon where employees previously tasked with lifting and stacking heavy objects are now finding roles that require them to operate and monitor robots that do the same. As technology progresses and more tasks become easier, awareness drives informing people of all the things technology can do for them can go a long way.
Human in the loop workflows
Competing perspectives coexist about automation, AI and the future of work, as mentioned in this article which states, "While some fear the end of employment and rising wealth inequality, others celebrate rising productivity and new frontiers for innovation and investment."
Human in the loop workflows is an attempt to bridge this gap between the two perspectives on how work might pan out for different people in the future. By using technology to help humans increase their productivity, automating repetitive and mundane tasks, organisations can free up valuable human resources for more important tasks, tasks that require thought and creativity.
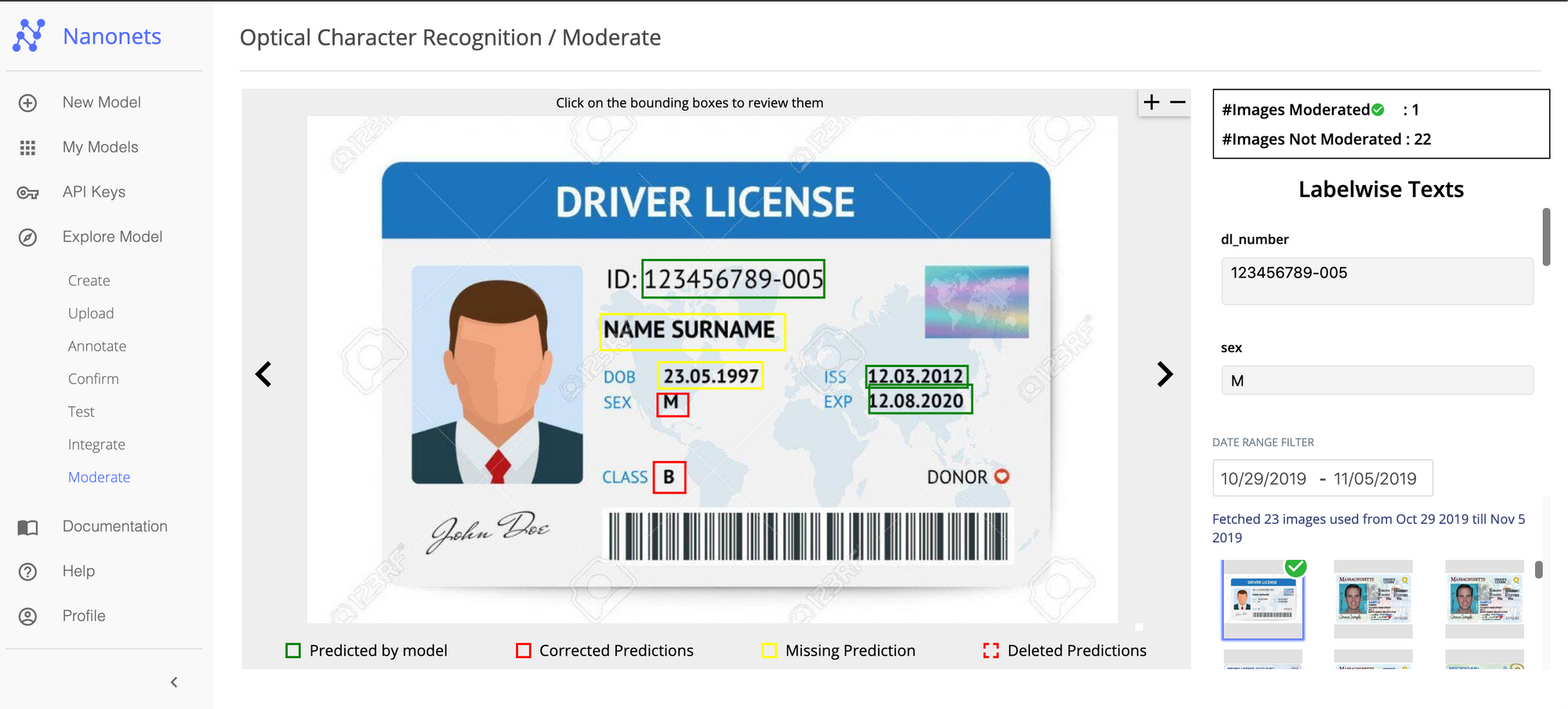
Implementing such a human-in-the-loop workflow in a digitization driven effort to transformation successfully involves us to be careful about the following things -
- Data gathering - Much time is spent by businesses gathering the right data that fits their use-case so that edge cases are handled gracefully and with minimal errors. This requires developers to scrape data off the web, gather data from several open-source resources and buying data from institutions and organisations.
- Data processing - This requires a lot of repetitive work where we need to get rid of or augment appropriately blurry images, noisy images, images that don't appear in the right orientation, consists of known fonts, etc. Following this cleaning, we also require a dedicated team of annotators that can generate good quality annotations for our data.
- Machine learning models - Finding the right architectures and methods to train your machine learning models that are able to function well at scale with a high accuracy can take time and extensive research. Understanding your data well is also just as important for a deep learning engineer trying to figure out the right algorithms to use.
- Correction - Once you have machine learning models that are able to generate predictions with an acceptable accuracy, it is not advisable always to have our models trained on more data and tuned better to serve a close to 100% automation goal. The lack of generalizability reduces the flexibility of these models and limits their reuse.
- Data entry - Once these predictions are cleaned and corrected, the data needs to be entered into a software which currently involves template and rule based solutions that are operated by people specifically hired for data entry. This sort of thing can be automated by building generalised models that can extract structure out of a document without explicit mention of the rules. This can aid the data entry procedures, make them faster, reduce fatigue related errors and enhance productivity.
Nanonets and digitization
We at Nanonets resolved to come up with a solution that takes care of all of the things mentioned above in our mission to make democratize machine learning.
OCR with Nanonets
The Nanonets OCR API allows you to build OCR models with ease. You can upload your data, annotate it, set the model to train and wait for getting predictions through a browser based UI without writing a single line of code, worrying about GPUs or finding the right architectures for your deep learning models. You can also acquire the JSON responses of each prediction to integrate it with your own systems and build machine learning powered apps built on state of the art algorithms and a strong infrastructure.
Using the GUI: https://app.nanonets.com/
You can also use the Nanonets-OCR API by following the steps below:
Step 1: Clone the Repo, Install dependencies
git clone https://github.com/NanoNets/nanonets-ocr-sample-python.git
cd nanonets-ocr-sample-python
sudo pip install requests tqdm
Step 2: Get your free API Key
Get your free API Key from https://app.nanonets.com/#/keys
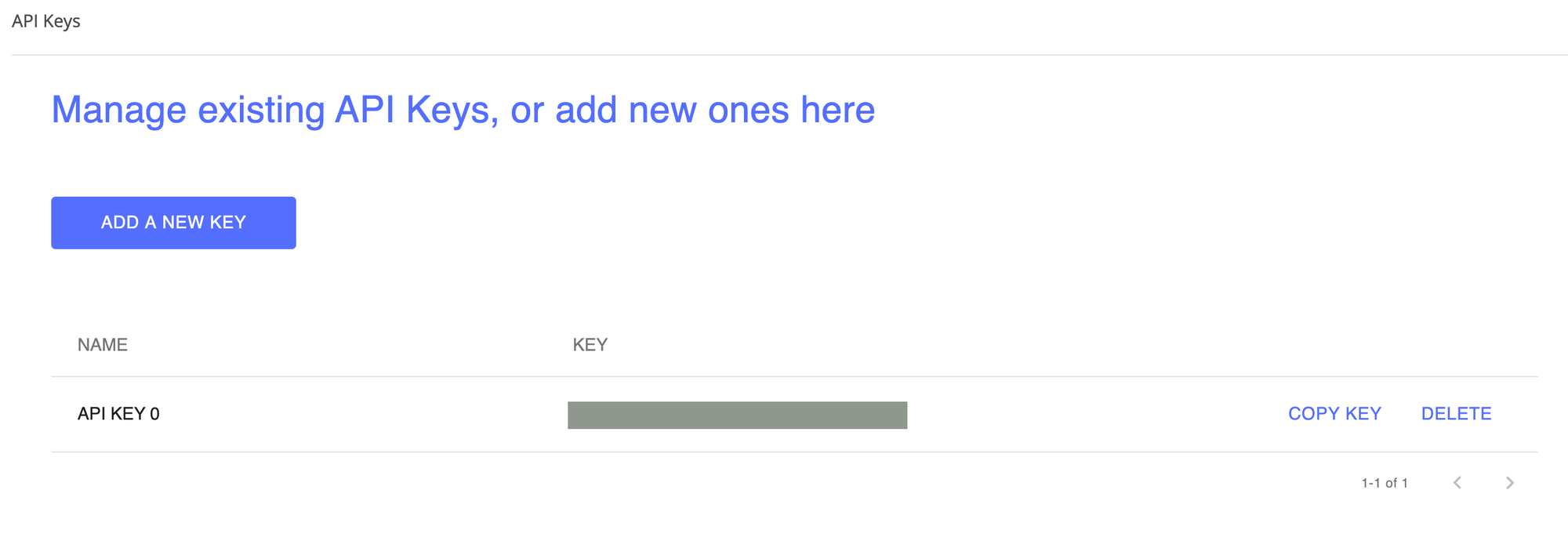
Step 3: Set the API key as an Environment Variable
export NANONETS_API_KEY=YOUR_API_KEY_GOES_HERE
Step 4: Create a New Model
python ./code/create-model.py
Note: This generates a MODEL_ID that you need for the next step
Step 5: Add Model Id as Environment Variable
export NANONETS_MODEL_ID=YOUR_MODEL_ID
Note: you will get YOUR_MODEL_ID from the previous step
Step 6: Upload the Training Data
The training data is found in images
(image files) and annotations
(annotations for the image files)
python ./code/upload-training.py
Step 7: Train Model
Once the Images have been uploaded, begin training the Model
python ./code/train-model.py
Step 8: Get Model State
The model takes ~2 hours to train. You will get an email once the model is trained. In the meanwhile you check the state of the model
python ./code/model-state.py
Step 9: Make Prediction
Once the model is trained. You can make predictions using the model
python ./code/prediction.py ./images/151.jpg
Nanonets and humans in the loop
The 'Moderate' screen aids the correction and entry processes and reduce the manual reviewer's workload by almost 90% and reduce the costs by 50% for the organisation.
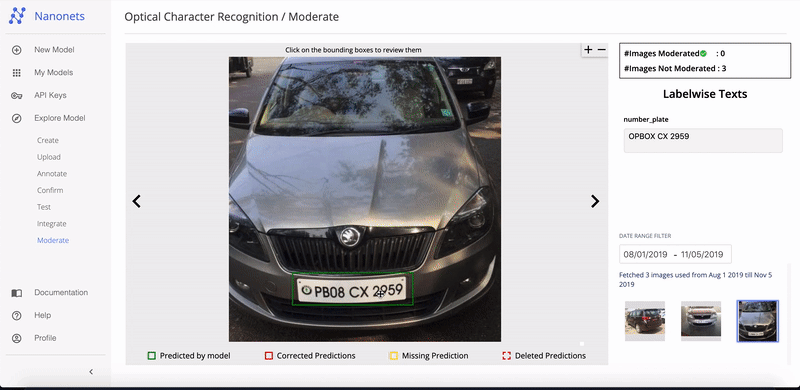
Features include
- Track predictions which are correct
- Track which ones are wrong
- Make corrections to the inaccurate ones
- Delete the ones that are wrong
- Fill in the missing predictions
- Filter images with date ranges
- Get counts of moderated images against the ones not moderated
All the fields are structured into an easy to use GUI which allows the user to take advantage of the OCR technology and assist in making it better as they go, without having to type any code or understand how the technology works.
Conclusion
We learnt about digital transformations, why they happen, what organisations can do to ensure a successful transformation. We also discussed the current direction technological advancement is heading in and how information in this age is driving innovation, what that means for society at large. We finally looked at how to tackle the problem of automation against employment numbers with human in the loop workflows.
Hope you enjoyed the article.