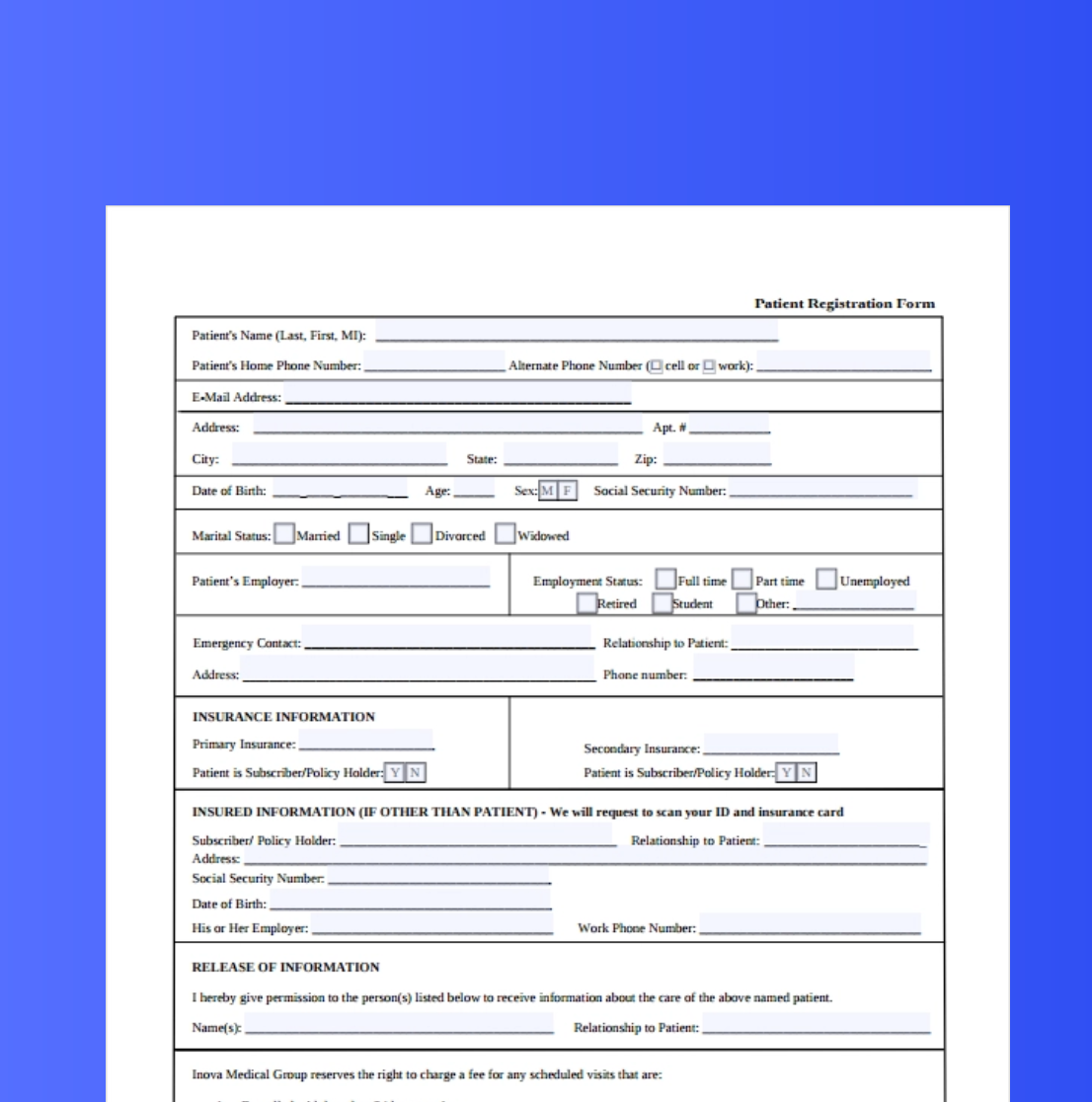
The healthcare industry accommodates a large amount of data, most of which is unstructured and complex. Personal health information has not been used to its full potential as the data available are fragmented and isolated.
But if this data could be extracted and organized correctly to create accurate and reliable information that could be utilized to achieve healthcare goals of early detection, delay the progression, and prevention of multiple diseases, reduction of high and growing healthcare costs, and improvement in patient communication to deliver an enhanced patient care overall.
Patient registration form and what does it contain?
A Patient Registration Form is a document filled out by a patient who visits a healthcare facility for the first time. It enables healthcare providers to gather personal and health-related information before registering them to receive their intended care.
The contents of a Patient Registration Form will vary among the healthcare institutions, but the general contents will be as follows.
The first section inquires about the patient’s details, including their name, sex, date of birth, address, marital status, contact information, and Identification Number in the form of a National Identity or Passport Number.
The second Section contains the information on personnel to be contacted in case of emergency, the next of kin, or the legal guardian of a minor.
The third section carries information on the insurance scheme of the patient, including the company name, insurance number, and policy.
The following section carries the patient consent form, including the patient declaration, confidentiality agreement, and other legally binding conditions, which should be signed with the patient's date.
Additionally, there are sections containing the medical history, current medications the patient is on, allergies, family history, history of substance abuse, etc.
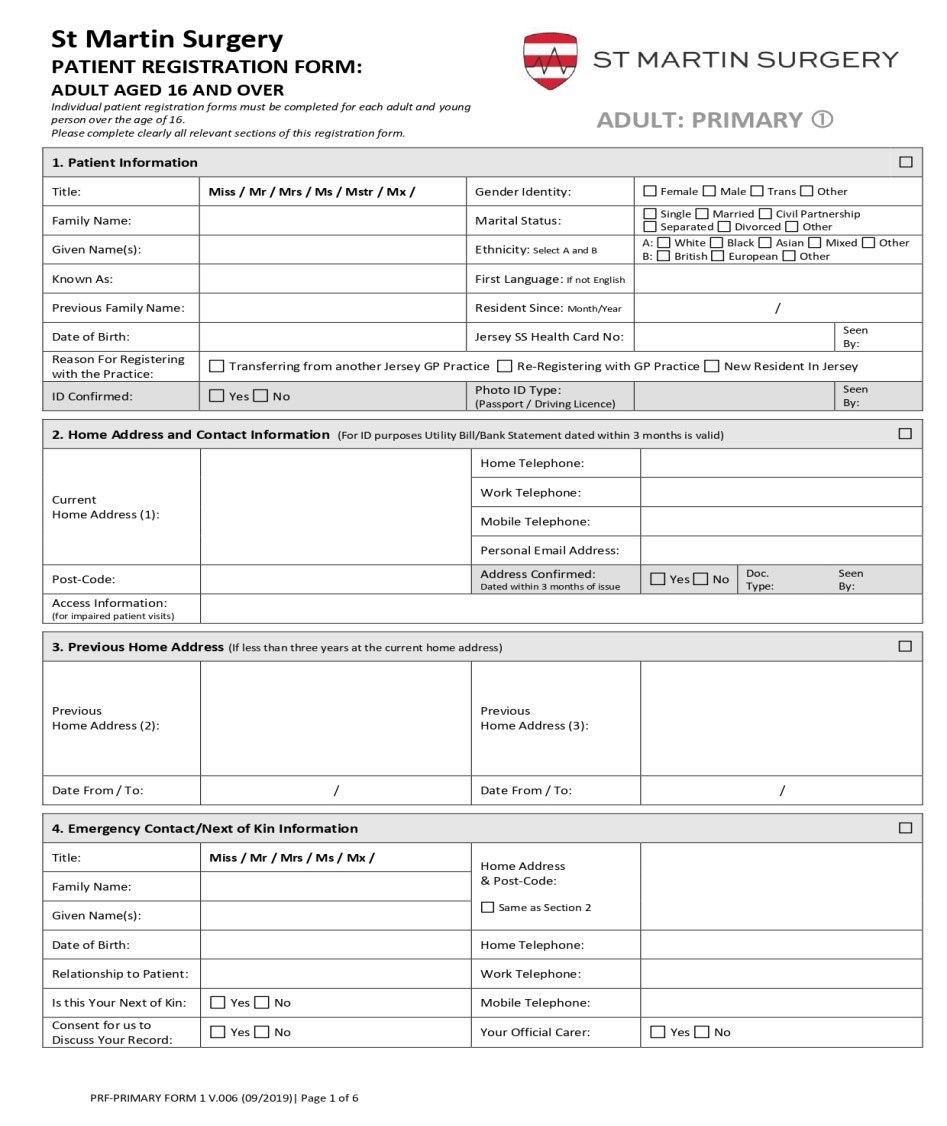
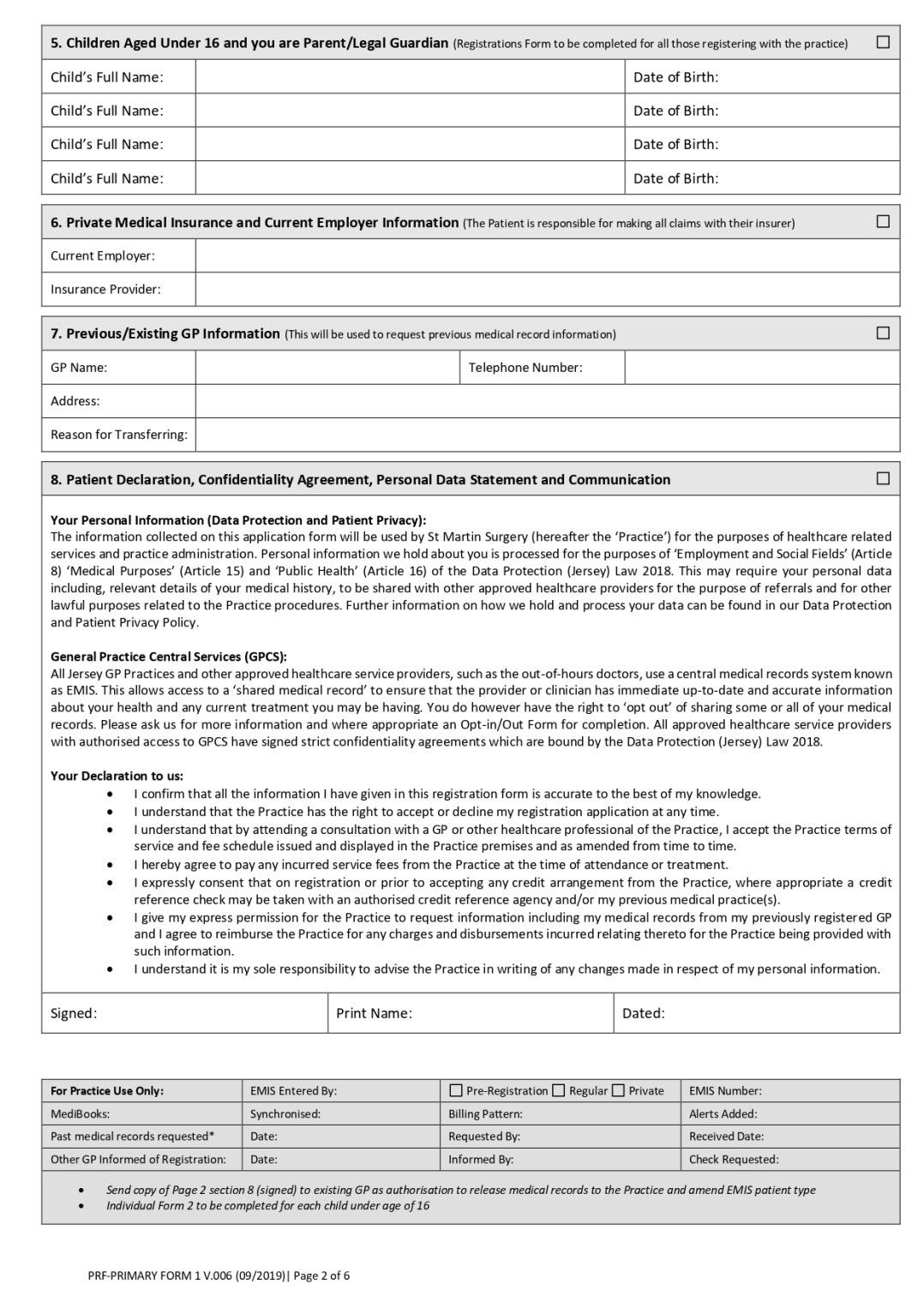
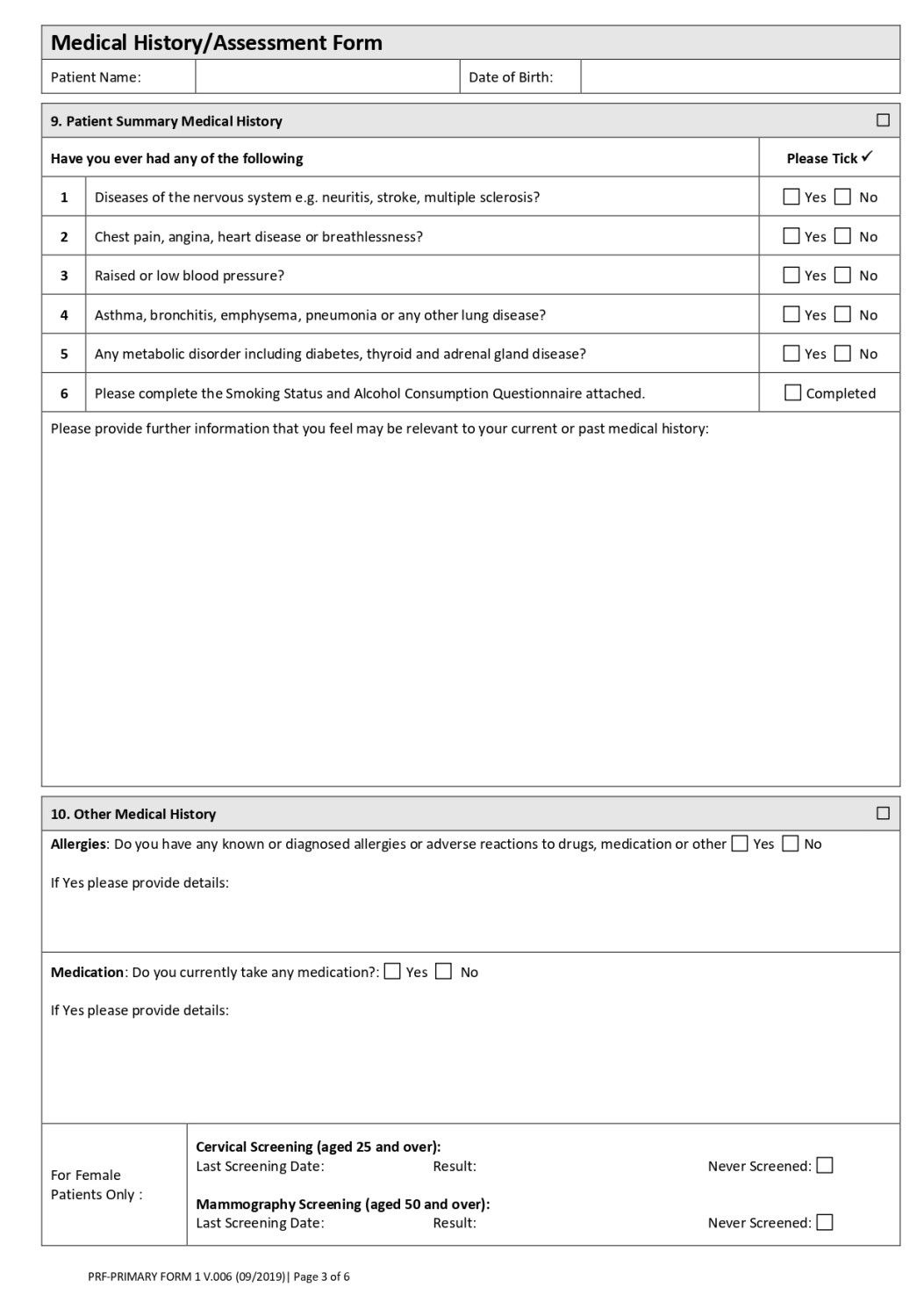
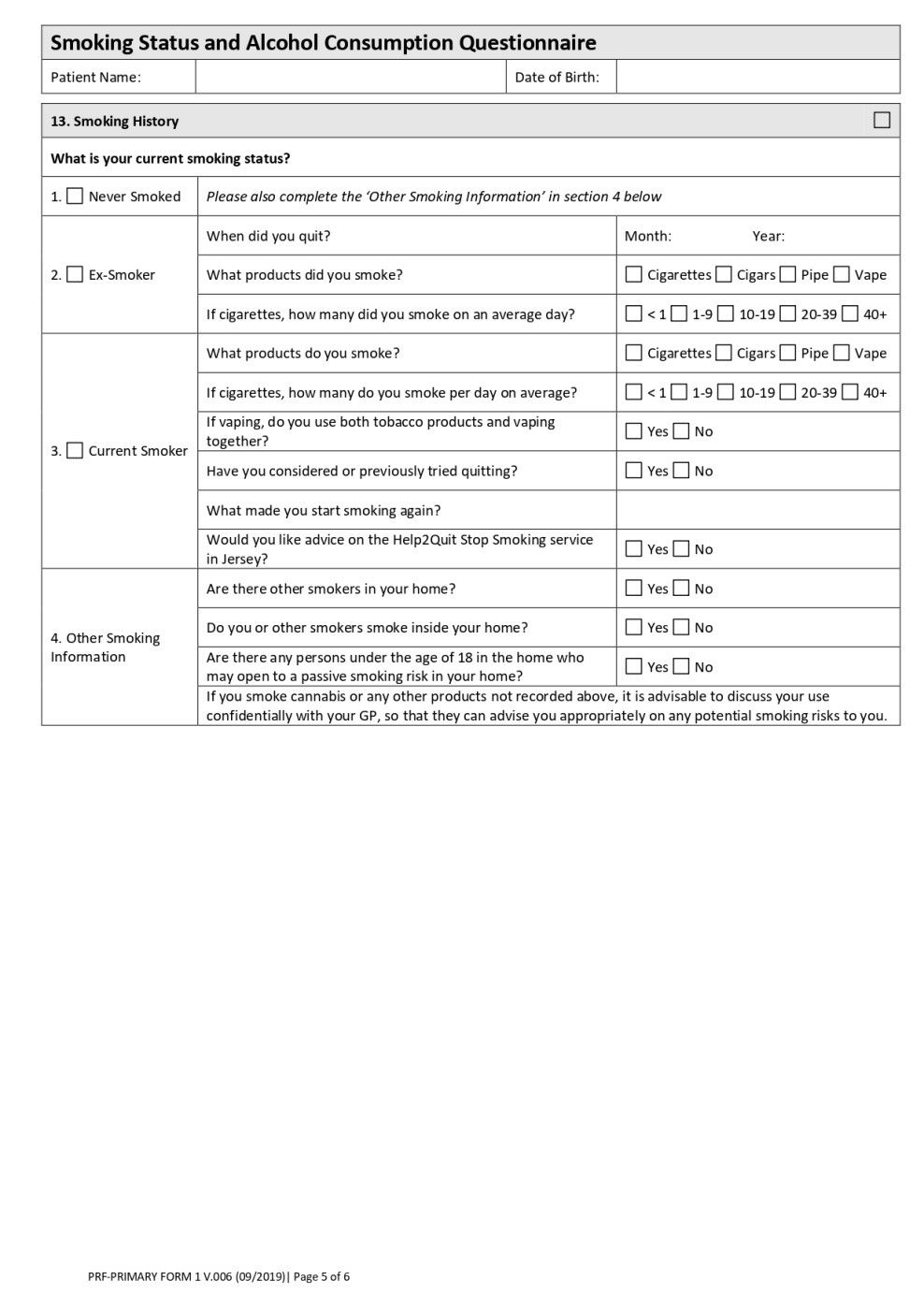
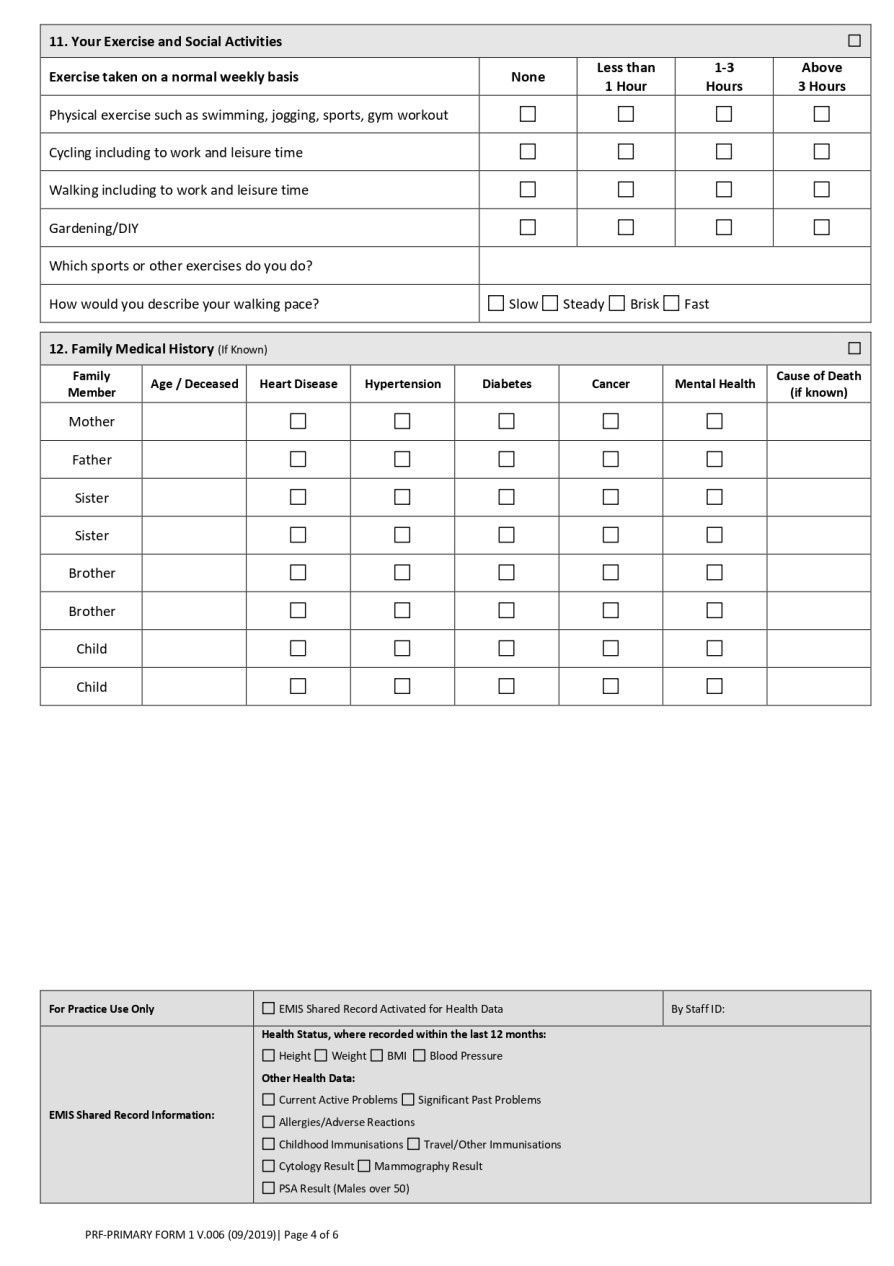
Current methods for data extraction from the patient registration forms
A. Manual data entry
In this method, an operator will manually feed the information in the patient registration form to a database. These traditional data entry methods depend on operator factors and will carry out more disadvantages than advantages compared to automated systems.
Pros
The capital expenditure will be less in terms of operator training and infrastructure as manual data entry does not require highly skilled staff and sophisticated software and hardware to compile and present the data.
Cons
As the health records are quite detailed, data extraction takes hours and could add errors to healthcare information during typing and calculations, by non-adherence to guidelines and definitions, and may result in non-uniformity in data. This could cause cascading effects resulting in poor diagnoses, erroneous prescriptions, and adverse patient outcomes.
Due to the complexity of extracted data, traditional methods only utilize a limited number of commonly collected variables for predictions. This can create false positives and false alarms on patients, which could result in alert fatigue, and clinically significant events will be missed, leading to poor patient management.
B. Electronic Health Records (EHR)
EHR captures a high volume of data, which is fragmented and isolated across many healthcare institutions, including hospitals, General Physician Practices, laboratories, pharmacies, etc.
Pros
EHR has reduced operator-level errors in data entry, calculations, and non-adherence to guidelines and data definitions, reducing medical errors. The quality of the care provided for the patient has improved, evidenced by a study done among United State physicians in 2011 showing that EHR has alerted 65% of possible medication errors and 62% of critical lab values, enhancing overall patient care by 78%.
Healthcare costs have been reduced through proper diagnoses, appropriate investigations, and management following accurate predictions made using EHR and deep learning techniques.
The usage of EHR enabled the process of Health Information Exchange (HIE), where patient-level information is shared among different organizations. This has created easy access for medical practitioners to one's medical records when patients seek medical assistance from healthcare providers at different locations.
Cons
Different health institutions have slightly different formats for presenting data. Meanwhile, the guidelines differ, and the diagnoses made through the International Classification of Diseases (ICD) may add random errors to EHR predictions. Therefore, not having uniform terminology, system architecture, and indexing may reduce the expected benefits from EHR.
EHR is associated with high start-up costs for hardware and operator training, which could be variable due to users' inequalities in computer literacy and database handling.
The confidentiality and security of patients' sensitive information are at stake as a large amount of data is gathered together, and proper security measures are not in place.
C. Hybrid approaches
As the information available in EHR are in the forms of non-standard codes and structures, health data transformation and loading approaches such as Dynamic ETL (Extraction, Transformation, and Loading) have come to practice to restructure and transform EHR data into a common format and standard terminologies to harmonize among different organizations and research data networks.
How to automate data extraction from medical reports?
Nanonets is an AI-based OCR software (GDPR & SOC2 complaint) that can automate medical document processing with no-code workflows.
Nanonets can automate multiple steps of healthcare document processing, including document upload, data extraction, data processing (data cleaning, formatting, conversion), approvals, and document archiving.
Nanonets adheres to your specific requirements, and being a completely no-code platform, it can be used by anyone in the organization.
Let’s delve into how you can use it to extract data from medical registration reports:
First, to use it, create a free account on Nanonets or log into your account.
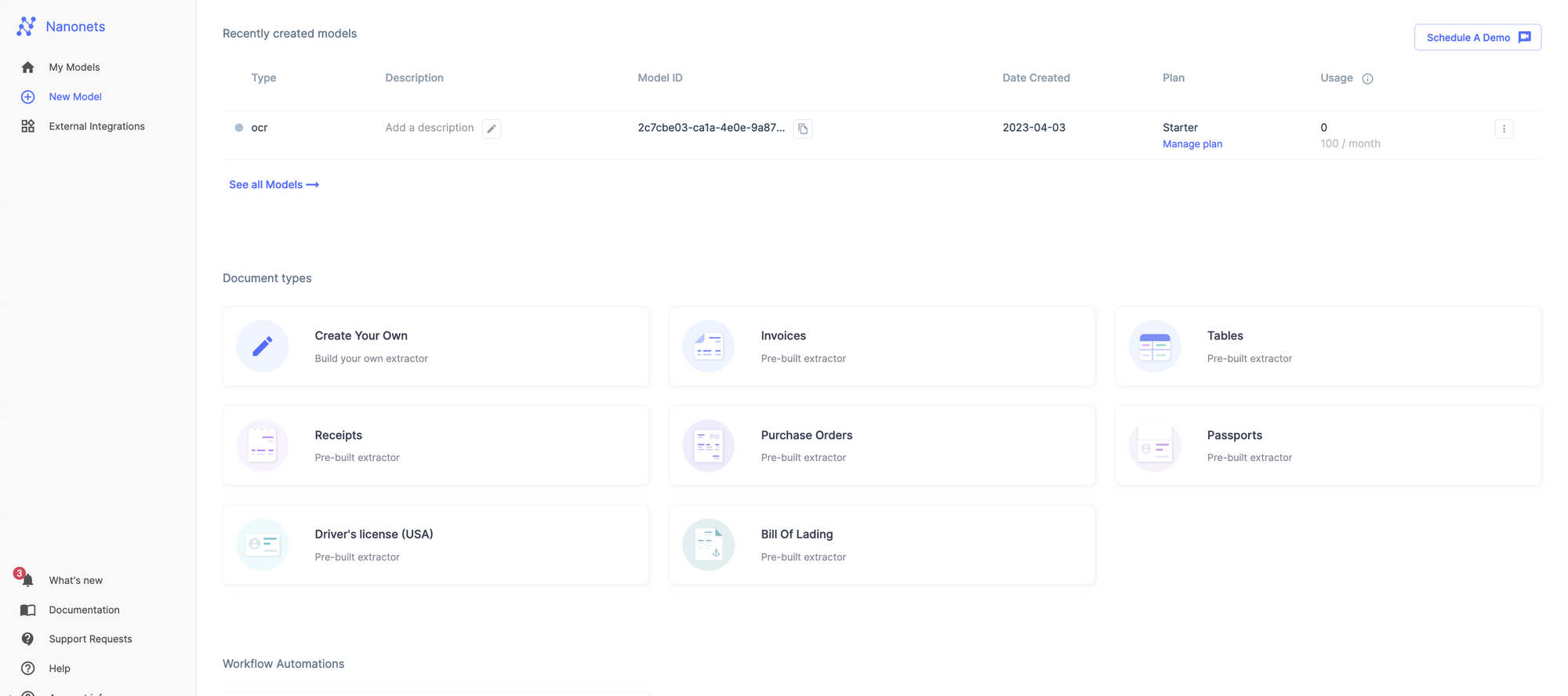
Select a custom OCR model. To train this model, you’ll have to provide ten medical reports.
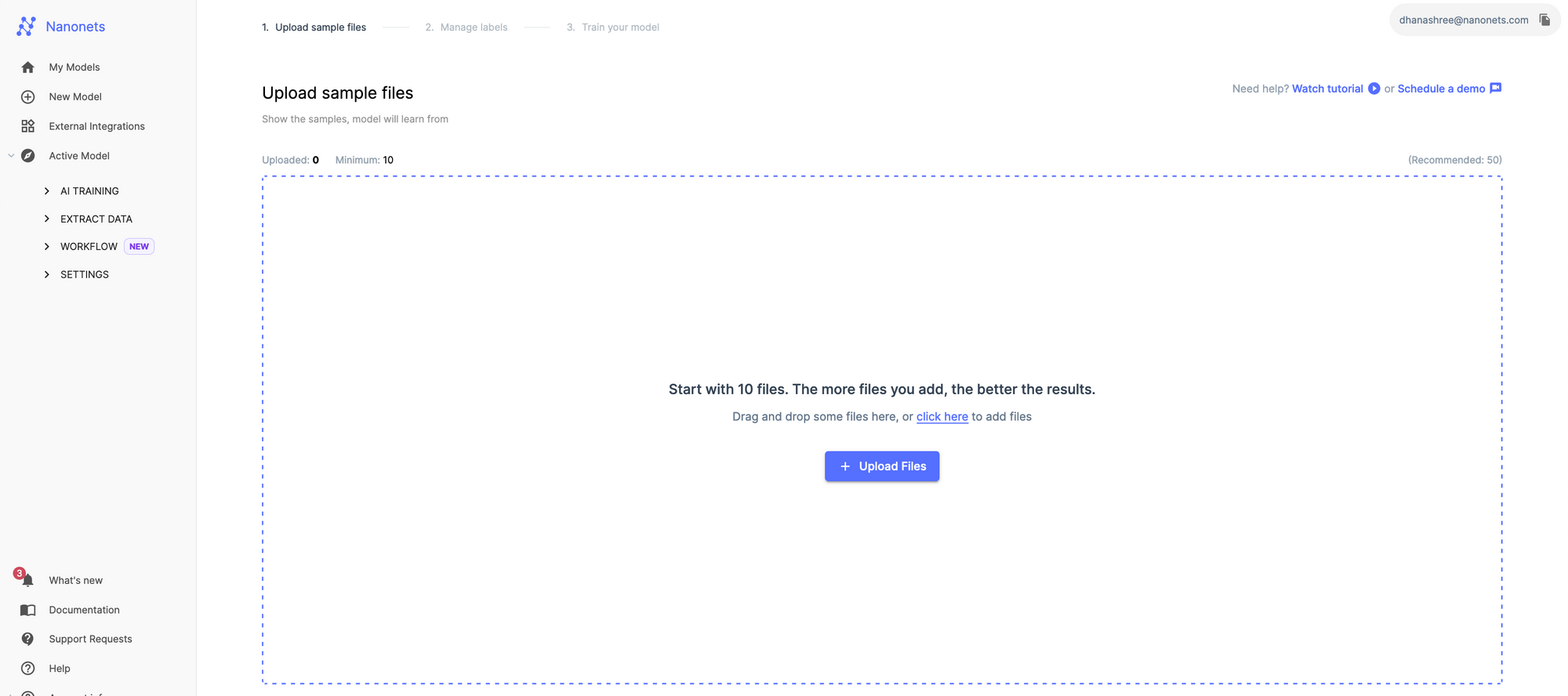
Once trained, you can now set up rules to format your data. You can change the number of zeros or look up the value in the database and more with these no-code rules.
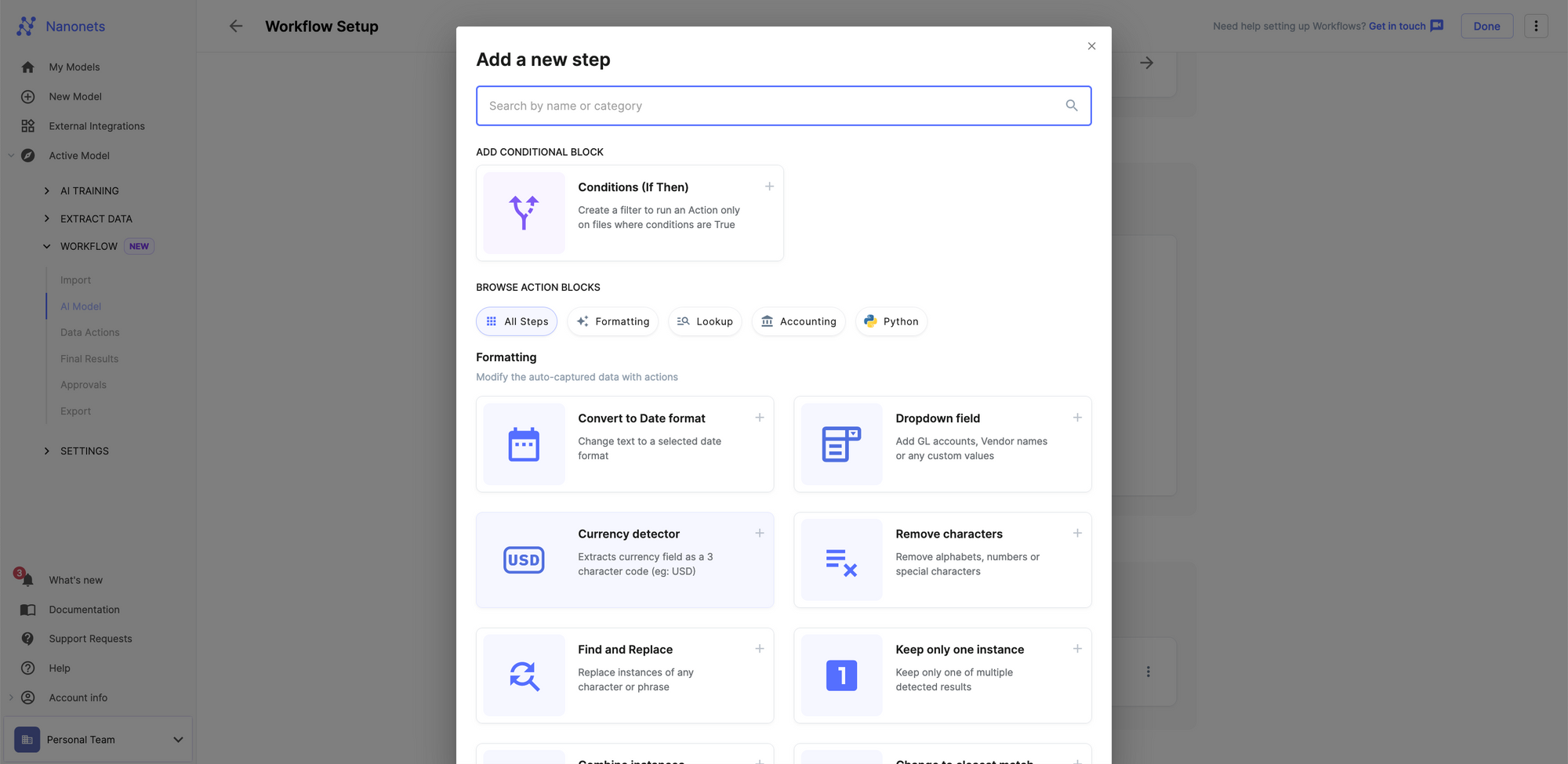
The next step is to export and select the way you want to export the data from your medical reports. Explore the options or select an integration and connect it directly to your healthcare EHR system.
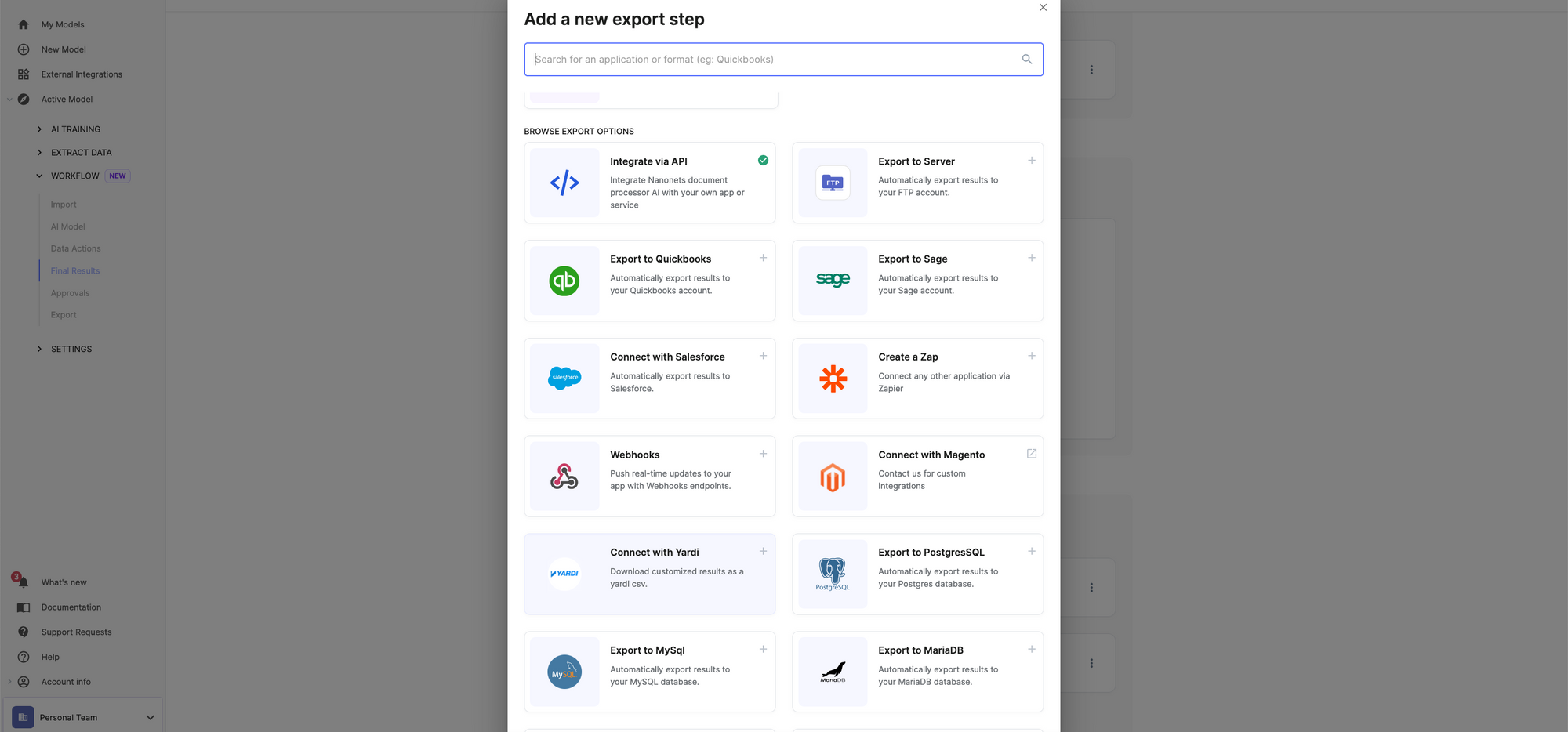
Need to do more? Set up a call with our AI experts where you can explain your use case to us, and we will set up workflows for you.
Why Nanonets?
Nanonets is an intelligent OCR platform. It doesn’t need a template to identify text from patient registration forms. It can identify text from an unrecognized document easily.
It’s easy to use, can be set up in 1 day, and ensures 99%+ accuracy during data extraction.
But apart from regular OCR features, here is what sets Nanonets apart:
Unparalleled Image Processing
Patient registration forms can have varying formats for different health institutions. Nanonets can handle data extraction from any document or image, which is not perfect to start with. With advanced pre and post-processing, the platform can deskew, reorient, rotate, crop, and perform fuzzy matching, so you get the exact data from your registration forms every time.
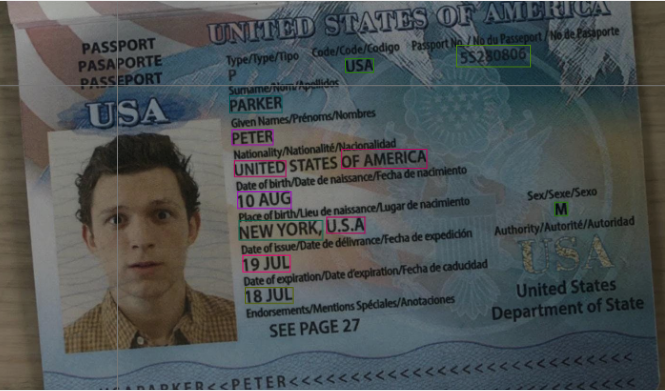
Best-in-class OCR
Nanonets can extract data from your medical document with over 98%+ accuracy. It can detect more than 40+ languages and supports custom OCR support.
Powerful integrations
You can automate data entry into your systems easily with Nanonets. Scan your documents and update patient profiles across 500+ business software in real-time with Nanonets integrations.
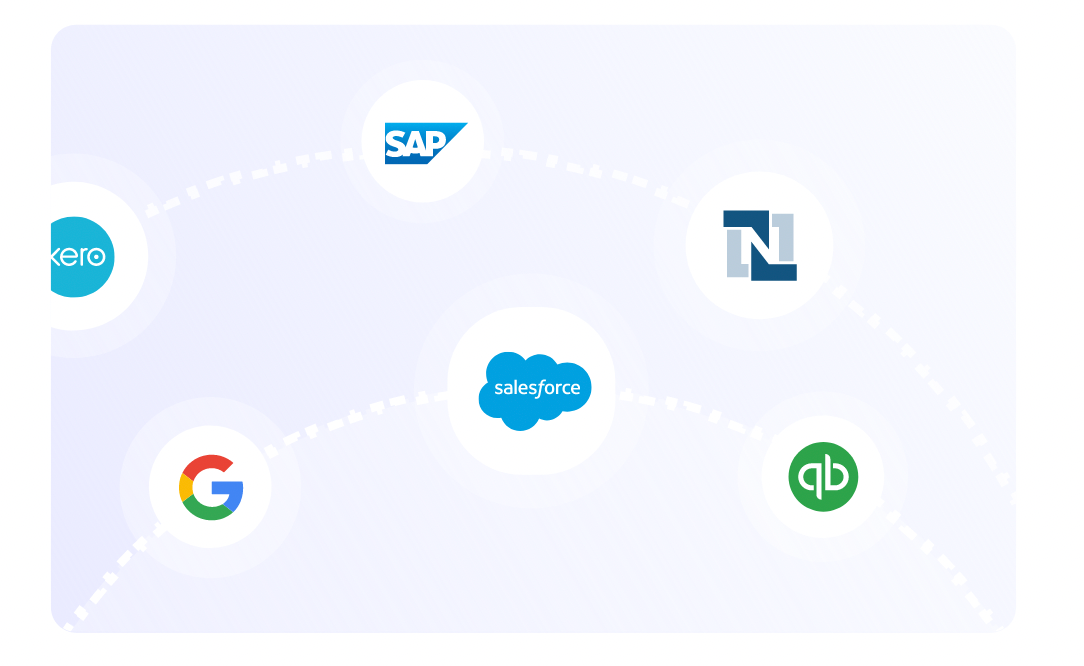
Automated customizable workflows
Automate document screening, patient onboarding, data formatting, data enrichment, medical report collection, data sync, document matching, and more with no-code workflows. Just punch in your rules and set it on autopilot mode.
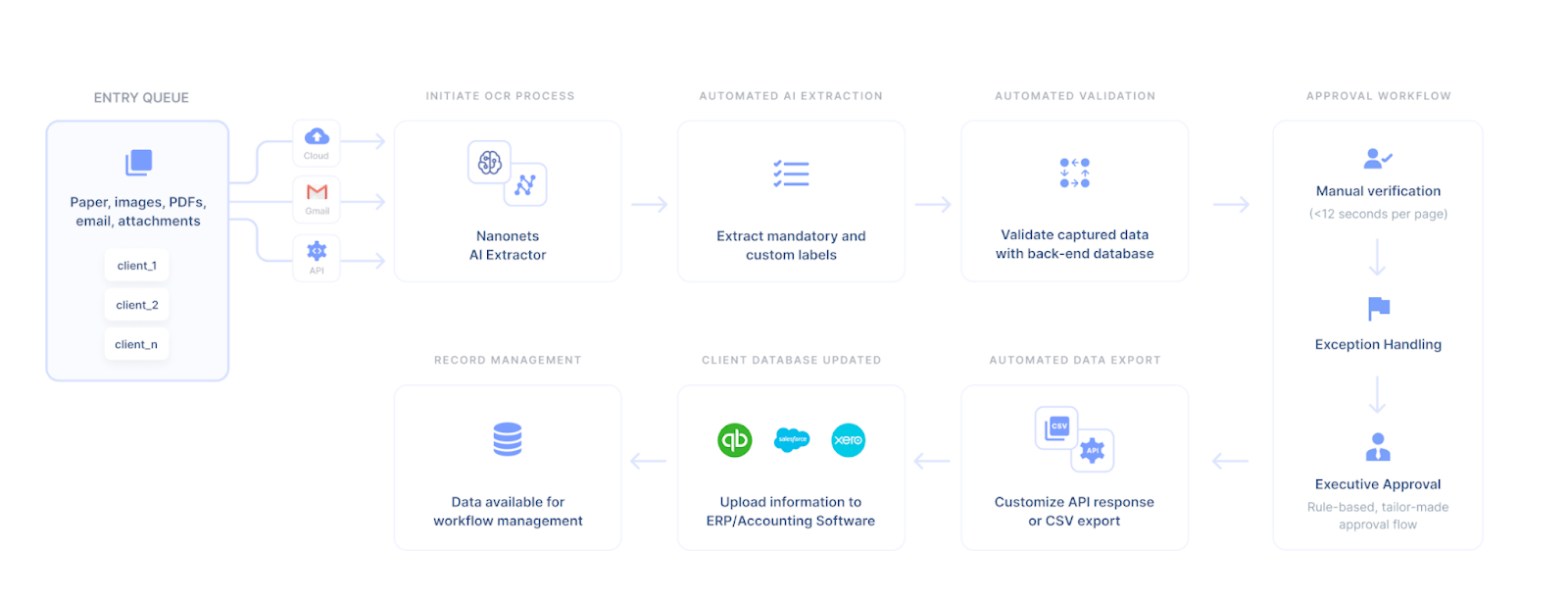
And more. Nanonets is customizable according to your needs and offers white-label OCR software and on-premise or cloud hosting options.
Do you need to extract data from patient registration forms?
If so, head to Nanonets or schedule a call with our team.
What are the limitations of current data extraction methods?
Technology
Health Information Management Systems that use EHR require costly network connections with high speed, reliable internet access, hardware, and software. Due to high start-up costs and the unavailability of affordable and effective technology, implementing Artificial Intelligence based methods of automated data extraction will only be a consistent program across some organizations.
Data Ownership
With the existing competitive relations among healthcare providers, problems arise regarding the type and amount of information exchanged. The proprietary information shared is limited on a 'read only' basis by the technology vendors. Therefore, up-to-date information will not be available.
Privacy concerns of patients
Since personal health information is dealt with, sharing information between organizations is only done for patient care abiding by privacy laws. Legal liabilities are associated to prevent unlawful disclosure of information; therefore, the risk of damage in data exchange should always outweigh potential rewards.
Why automate data extraction from patient registration forms?
A. Improved data accuracy
Rather than slow, error-prone traditional data entry methods which waste valuable employee talent, automated data extraction ensures greater accuracy with repeated use.
As data extraction from EHR and free texts are incorporated into deep learning techniques, valid and accurate predictions are made over divergent healthcare domains concerning the quality and outcomes of care and utilization of resources. Reliable and accurate information will help in correct diagnoses and appropriate management, enhancing patient outcomes.
B. Increased efficiency
The automated systems will bring together the fragmented and isolated personal health information, which has yet to be utilized to its full potential, to a structured form improving the effectiveness and efficiency of the care provided.
A Study done in 2016 revealed data analysts spend only 20% of their work hours on data analysis while the rest of the time is spent on gathering and extracting the data. Automated data extraction reduces the workforce and time wasted on manual error-prone data extraction and directs them to enhance patient care.
C. Enhanced patient care
People will access healthcare facilities from different locations. Therefore, an interconnected and automated system will provide healthcare providers with a clear picture of the patient's condition, and consistent and effective management could be offered. 30 - 50% of United States Physicians have reported that electronic systems are beneficial in providing recommended care and appropriate investigations and allow good patient communication through enhanced overall patient care in 78% of a study population.
D. Reduced costs
As patient records provide a multitude of data on different domains, manual data entry will be time-consuming and costly with a poorly valued erroneous outcome. Even though automated data extraction has a high start-up cost, in the long run, cost reduction could be achieved when regular repetitive activities consuming human labor could be automated to gain structured and accurate data and predictions.
As opposed to isolated data collection, automated data extraction and compilation will provide centrally controlled databases of personal health information that could be used among many health care providers, reducing data duplication costs.
E. Streamlined workflow and decision-making
EHR based on Fast Healthcare Interoperability Resources (FHIR) and deep learning methods can provide accurate predictions on medical events in multiple centers. Predictions are done on mortality rates, readmissions, length of hospital stay, etc. which will help to manage the available resources to reach the demand. The un-/semi-structured data extracted from a patient registration form could be utilized to identify the effects and shortcomings of the treatments and comorbidities and to determine the expected outcome in the patient with a particular condition.